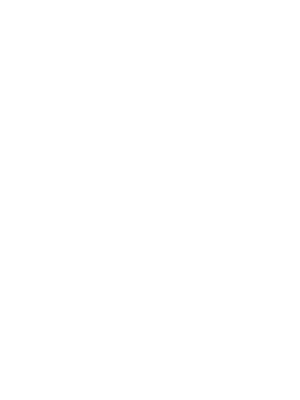
• dr Thi Thuy Linh Nguyen
Position: adiunkt
Unit: Instytut Nauk o Ziemi
Adress: 41-200 Sosnowiec, ul. Będzińska 60
Floor: II
Room: 203
Phone: (32) 3689 684
E-mail: thi-thuy-linh.nguyen@us.edu.pl
Publications list: Publications by CINiBA
Publications list: Publications by OPUS
Scopus Author ID: 57668633200
Publications from the Scopus database
2024
Aiyelokun, O.; Pham, Q. B.; Aiyelokun, O.; Linh, N. T. T.; Roy, T.; Anh, D. Tran; Łupikasza, E. B.
In: Pure and Applied Geophysics, vol. 181, no. 5, pp. 1725-1744, 2024, ISSN: 00334553, (2).
@article{2-s2.0-85191714250,
title = {Effectiveness of Integrating Ensemble-Based Feature Selection and Novel Gradient Boosted Trees in Runoff Prediction: A Case Study in Vu Gia Thu Bon River Basin, Vietnam},
author = { O. Aiyelokun and Q.B. Pham and O. Aiyelokun and N.T.T. Linh and T. Roy and D. Tran Anh and E.B. Łupikasza},
url = {https://www.scopus.com/inward/record.uri?eid=2-s2.0-85191714250&doi=10.1007%2fs00024-024-03486-0&partnerID=40&md5=aa3029c673e350dcb8ceca66f6245985},
doi = {10.1007/s00024-024-03486-0},
issn = {00334553},
year = {2024},
date = {2024-01-01},
journal = {Pure and Applied Geophysics},
volume = {181},
number = {5},
pages = {1725-1744},
publisher = {Birkhauser},
abstract = {Traditional rainfall-runoff modeling techniques require large datasets and often an exhaustive calibration process, which is challenging, especially in poorly-gauged basins and resource-limited settings. Therefore, it is necessary to examine new ways of constructing predictive models for runoff that can achieve satisfactory results, while also minimizing the data requirement and model construction time. In this study, the effectiveness of integrating the Random Forest (RF) as an important feature identifier with novel gradient boosted trees to achieve satisfactory results was examined for two adjacent catchments in Vietnam. Antecedent daily runoff in combination with daily and one-day antecedent rainfall was found to significantly influence the runoff at the outlet of the catchments. Categorical Boosting (CatBoost) and Extreme Gradient Boosting (XGBoost) were effective in predicting day-ahead runoff. For instance, CatBoost with NSE, d, r, and R2 values of 0.92, 0.98, 0.96, and 0.92, respectively, and XGBoost with NSE, d, r, and R2 values of 0.91, 0.98, 0.96, and 0.92, respectively, are well suited for predicting runoff. A comparative analysis of their results with previous studies revealed that the models were very effective since they were able to better reduce generalization errors at different calibration and validation phases. This study presents the integration of RF and gradient boosted trees as a simplified alternative to computationally expensive and data-intensive physically-based rainfall-runoff models. The practitioners can build upon the experimentation presented in this study to minimize the computational time requirement, construction process complexity, and data requirement, which are often serious constraints in physically-based rainfall-runoff modeling. © The Author(s), under exclusive licence to Springer Nature Switzerland AG 2024.},
note = {2},
keywords = {},
pubstate = {published},
tppubtype = {article}
}
2023
Asmoay, A. S.; Mohamed, A.; Alshehri, F.; Linh, N. T. T.; Al-Ansari, N.; Othman, A.
Water quality assessment in dry regions using statistical methods Journal Article
In: Journal of King Saud University - Science, vol. 35, no. 5, 2023, ISSN: 10183647.
@article{2-s2.0-85152107776,
title = {Water quality assessment in dry regions using statistical methods},
author = { A.S. Asmoay and A. Mohamed and F. Alshehri and N.T.T. Linh and N. Al-Ansari and A. Othman},
url = {https://www.scopus.com/inward/record.uri?eid=2-s2.0-85152107776&doi=10.1016%2fj.jksus.2023.102665&partnerID=40&md5=9e8a3961a493b82c7b11fe6914f4238c},
doi = {10.1016/j.jksus.2023.102665},
issn = {10183647},
year = {2023},
date = {2023-01-01},
journal = {Journal of King Saud University - Science},
volume = {35},
number = {5},
publisher = {Elsevier B.V.},
abstract = {Water demands have increased even more in recent decades because of the high population density. Surface and groundwater resources are insufficient to meet these demands. As a result, governments have turned to the treatment of sewage water. Sewage water contains multiple types of contamination, creating a major health risk. In the research region, 48 water samples were obtained, including 18 samples of surface water and 30 samples of groundwater. The Canadian Council Water Quality Index (CCWQI) program calculates the water quality index to evaluate the water quality for drinking and human use. The World Health Organization (WHO) and the Egyptian Ministry of Health (EMH) determined regulatory limits for drinking water and each value of the investigated parameter connected with them. According to the findings, 79% of the tested water samples are safe to drink and are excellent for human and wildlife use. Due to infiltration or recharging of groundwater with drainage water, as well as the involvement of dissolution, leaching processes, and anthropogenic activities that damage human health, animals, and some plants, these samples are unfit for drinking and domestic consumption. The heavy metal level of Cd and Pb in the examined water samples was found to be above WHO and EMH acceptable limits. Furthermore, due to oral exposures, the examined water samples may cause complex health concerns such as non-carcinogenic and carcinogenic influences for children over adults due to a reduction in children's immunity. As a result, water treatment should be carried out in the examined region to protect the health of the residents. © 2023 The Author(s)},
keywords = {},
pubstate = {published},
tppubtype = {article}
}
Emami, S.; Dehghanisanij, H.; Achite, M.; Al-Ansari, N.; Linh, N. T. T.
Application of ANFIS, ELM, and ANN models to assess water productivity indicators based on agronomic techniques in the Lake Urmia Basin Journal Article
In: Applied Water Science, vol. 13, no. 2, 2023, ISSN: 21905487.
@article{2-s2.0-85145358321,
title = {Application of ANFIS, ELM, and ANN models to assess water productivity indicators based on agronomic techniques in the Lake Urmia Basin},
author = { S. Emami and H. Dehghanisanij and M. Achite and N. Al-Ansari and N.T.T. Linh},
url = {https://www.scopus.com/inward/record.uri?eid=2-s2.0-85145358321&doi=10.1007%2fs13201-022-01851-9&partnerID=40&md5=e18305980a1342f69c16ab950dc236ef},
doi = {10.1007/s13201-022-01851-9},
issn = {21905487},
year = {2023},
date = {2023-01-01},
journal = {Applied Water Science},
volume = {13},
number = {2},
publisher = {Springer Science and Business Media Deutschland GmbH},
abstract = {Water productivity (WP) is one of the most important critical indicators in the essential planning of water consumption in the agricultural sector. For this purpose, the WP and economic water productivity (WPe) were estimated using agronomic technologies. The impact of agronomic technologies on WP and WPe was carried out in two parts of field monitoring and modeling using novel intelligent approaches. Extreme learning machine (ELM), adaptive neuro-fuzzy inference system (ANFIS), and artificial neural network (ANN) methods were used to model WP and WPe. A dataset including 200 field data was collected from five treatment and control sections in the Malekan region, located in the southeast of Lake Urmia, Iran, for the crop year 2020–2021. Six different input combinations were introduced to estimate WP and WPe. The models used were evaluated using mean squared error (RMSE), relative mean squared error (RRMSE), and efficiency measures (NSE). Field monitoring results showed that in the treatment fields, with the application of agronomic technologies, the crop yield, WP, and WPe increased by 17.9%, 30.1%, and 19.9%, respectively. The results explained that irrigation water in farms W1, W2, W3, W4, and W5 decreased by 23.9%, 21.3%, 29.5%, 16.5%, and 2.7%, respectively. The modeling results indicated that the ANFIS model with values of RMSE = 0.016},
keywords = {},
pubstate = {published},
tppubtype = {article}
}
Anh, D. Tran; Pandey, M.; Mishra, V. N.; Singh, K. K.; Ahmadi, K.; Janizadeh, S.; Tran, T. T.; Linh, N. T. T.; Dang, N. M.
In: Applied Soft Computing, vol. 132, 2023, ISSN: 15684946, (2).
@article{2-s2.0-85143834749,
title = {Assessment of groundwater potential modeling using support vector machine optimization based on Bayesian multi-objective hyperparameter algorithm},
author = { D. Tran Anh and M. Pandey and V.N. Mishra and K.K. Singh and K. Ahmadi and S. Janizadeh and T.T. Tran and N.T.T. Linh and N.M. Dang},
url = {https://www.scopus.com/inward/record.uri?eid=2-s2.0-85143834749&doi=10.1016%2fj.asoc.2022.109848&partnerID=40&md5=0707f0fb3625f21923aea68081a381fc},
doi = {10.1016/j.asoc.2022.109848},
issn = {15684946},
year = {2023},
date = {2023-01-01},
journal = {Applied Soft Computing},
volume = {132},
publisher = {Elsevier Ltd},
abstract = {Today, water supply in order to achieve sustainable development goals is one of the most important concerns and challenges in most countries. For this reason, accurate identification of areas with groundwater potential is one of the important tools in the protection, management and exploitation of water resources. Accordingly, the present study was conducted with the aim of modeling and predicting groundwater potential in Markazi province, Iran using Multivariate adaptive regression spline (MARS) and Support vector machine (SVM) machine learning models and using two random search (RS) and Bayesian optimization hyperparameter algorithms to optimize the parameters of the SVM model. For this purpose, 18 variables affecting the groundwater potential and 3482 spring locations were used to model the groundwater potential. Data for modeling were divided into two categories of training (70%) and validation (30%). The receiver operating characteristics (ROC) were used to evaluate the performance of the models. The results of evaluation models showed that using hyperparameters random search and Bayesian optimization were improved SVM accuracy in training and validation stages. Bayesian optimization methods are very efficient because they are consciously choosing the parameters of the model that this strategy improves the performance of the model. Evaluating accuracy in the validation stage showed that the AUC value is for MARS, SVM, RS-SVM and B-SVM models 87.40%, 88.25%, 90.73% and 91.73%, respectively. The results of assessment variables importance showed elevation, precipitation in the coldest month, soil and slope variables have the most importance in modeling groundwater potential, while aspect, profile curvature and TWI variables, have the least importance in predicting groundwater potential in Markazi province. © 2022 Elsevier B.V.},
note = {2},
keywords = {},
pubstate = {published},
tppubtype = {article}
}
Nazaripouya, H.; Sepehri, M.; Atapourfard, A.; Ghermezcheshme, B.; Santos, Celso A. G.; Khoshbakht, M.; Meshram, S. G.; Rana, V. K.; Linh, N. T. T.; Pham, Q. B.; Anh, D. Tran
Evaluating Sediment Yield Response to Watershed Management Practices (WMP) by Employing the Concept of Sediment Connectivity Journal Article
In: Sustainability (Switzerland), vol. 15, no. 3, 2023, ISSN: 20711050, (1).
@article{2-s2.0-85147861288,
title = {Evaluating Sediment Yield Response to Watershed Management Practices (WMP) by Employing the Concept of Sediment Connectivity},
author = { H. Nazaripouya and M. Sepehri and A. Atapourfard and B. Ghermezcheshme and Celso A.G. Santos and M. Khoshbakht and S.G. Meshram and V.K. Rana and N.T.T. Linh and Q.B. Pham and D. Tran Anh},
url = {https://www.scopus.com/inward/record.uri?eid=2-s2.0-85147861288&doi=10.3390%2fsu15032346&partnerID=40&md5=90ff2900e8bea3a58f0a62f723482c7b},
doi = {10.3390/su15032346},
issn = {20711050},
year = {2023},
date = {2023-01-01},
journal = {Sustainability (Switzerland)},
volume = {15},
number = {3},
publisher = {MDPI},
abstract = {Watershed management practices (WMP) are widely used in catchments as a measure to reduce soil erosion and sediment-related problems. We used a paired catchment in the Gonbad region of Hamadan province, Iran, to evaluate sediment yield response to watershed management practices (WMP) by employing the concept of sediment connectivity (SC). To do this, the SC index as a representation of sediment yield was firstly simulated for the control catchment that there is no WMP. In the next step, the SC index was simulated for impacted catchment, including some WMP, i.e., seeding, pit-seeding, and exclosure. After assessing the accuracy of the produced SC maps using filed observations and erosion plots, the SC maps using quantile-quantile plot (Q-Q plot) were compared to achieve the role of WMP in reducing the rate of sediment yield. The Q-Q plot showed that there is a strong similarity between the SC of catchments, it can be concluded that the WMP has no significant impact on the reducing rate of the sediment yield in this study. © 2023 by the authors.},
note = {1},
keywords = {},
pubstate = {published},
tppubtype = {article}
}
Polong, F.; Deng, K. A. K.; Pham, Q. B.; Linh, N. T. T.; Abba, S. I.; Ahmed, A. N.; Anh, D. Tran; Khedher, K. M.; El-Shafie, A.
Separation and attribution of impacts of changes in land use and climate on hydrological processes Journal Article
In: Theoretical and Applied Climatology, vol. 151, no. 3-4, pp. 1337-1353, 2023, ISSN: 0177798X, (3).
@article{2-s2.0-85146394020,
title = {Separation and attribution of impacts of changes in land use and climate on hydrological processes},
author = { F. Polong and K.A.K. Deng and Q.B. Pham and N.T.T. Linh and S.I. Abba and A.N. Ahmed and D. Tran Anh and K.M. Khedher and A. El-Shafie},
url = {https://www.scopus.com/inward/record.uri?eid=2-s2.0-85146394020&doi=10.1007%2fs00704-022-04351-7&partnerID=40&md5=ed1fbe7abde077732e2aecd3dfdf5f8b},
doi = {10.1007/s00704-022-04351-7},
issn = {0177798X},
year = {2023},
date = {2023-01-01},
journal = {Theoretical and Applied Climatology},
volume = {151},
number = {3-4},
pages = {1337-1353},
publisher = {Springer},
abstract = {This study aims to assess, compare, and attribute the effects due to separate and combined land use/land cover (LULC) and climate changes on hydrological processes in a tropical catchment. The Soil and Water Assessment Tool (SWAT) model is set up and calibrated for a small contributing sub-basin of the Tana River Basin (TRB) in Kenya. The model is then applied to simulate the hydrological components (i.e.; streamflow (FLOW); evapotranspiration (ET); soil water (SW); and water yield (WYLD)) for different combinations of LULC and climate scenarios. Land use data generated from Land Satellite 5 Thematic Mapper (Landsat 5TM) images for two different periods (1987 and 2011) and satellite-based precipitation data from the African Rainfall Climatology version 2 (ARC2) dataset are utilized as inputs to the SWAT model. The Nash–Sutcliffe model efficiency (NSE), coefficient of determination (R2), percent bias (PBIAS), and the ratio of root mean square error to the standard deviation (RSR) for daily streamflow were 0.73, 0.76, 3.16%, and 0.51 in calibration period, respectively, and 0.45, 0.54, 12.53%, and 0.79 in validation period, respectively, suggesting that the model performed relatively good. An analysis of the LULC data for the catchment showed that there was an increase in agricultural, grassland, and forested land with a concomitant decrease in woodland and shrubland. Simulation results revealed that change in climate had a more significant effect on the simulated parameters than the change in LULC. It is shown that changes in LULC only had very minor effects in the simulated parameters. The monthly mean FLOW and WYLD decreased by 0.02% and 0.11%, respectively, while ET and SW increased by a monthly mean of 0.2% and 2.2%. Varying the catchment climate and holding the land use constant reduced FLOW, ET, SW, and WYLD by an average monthly mean of 43.2%, 21%, 13%, and 70%, respectively, indicating that climate changes have more significant effects on the catchment hydrological processes than changes in LULC. Thus, it is necessary to evaluate and identify the isolated and combined effects of LULC and climatic changes when assessing impacts on the TRB’s hydrological processes. © 2022, The Author(s), under exclusive licence to Springer-Verlag GmbH Austria, part of Springer Nature.},
note = {3},
keywords = {},
pubstate = {published},
tppubtype = {article}
}
2022
Kumar, Dh.; Bhardwaj, D. R.; Sharma, P.; Bharti,; Sankhyan, N.; Al-Ansari, N.; Linh, N. T. T.
In: Forests, vol. 13, no. 10, 2022, ISSN: 19994907, (2).
@article{2-s2.0-85140777485,
title = {Population Dynamics of Juniperus macropoda Bossier Forest Ecosystem in Relation to Soil Physico-Chemical Characteristics in the Cold Desert of North-Western Himalaya},
author = { Dh. Kumar and D.R. Bhardwaj and P. Sharma and Bharti and N. Sankhyan and N. Al-Ansari and N.T.T. Linh},
url = {https://www.scopus.com/inward/record.uri?eid=2-s2.0-85140777485&doi=10.3390%2ff13101624&partnerID=40&md5=633498978d93df4bb147f86a25ff1d56},
doi = {10.3390/f13101624},
issn = {19994907},
year = {2022},
date = {2022-01-01},
journal = {Forests},
volume = {13},
number = {10},
publisher = {MDPI},
abstract = {Juniperus macropoda is the only tree species of a cold desert ecosystem that is experiencing high anthropogenic pressure and has a poor regeneration status due to harsh environmental conditions. Due to the limited distribution of Juniperus macropoda in this region, the species have remained largely unexplored in terms of understanding the distribution pattern along the elevation and soil fertility gradients. Therefore, the current research was carried out along the elevational gradient, starting from the base line at 3000 m above sea level (m asl) asl with an elevational plot distance of 180 m. The study revealed that the average density of J. macropoda declined gradually from the first elevation range, i.e., 3000–3180 m asl onward, and extended up to the elevation range of 3900–4080 m asl. However, the average seedling and sapling densities were highest at mid-elevation and extended up to an elevation range of 4080–4260 m asl. The J. macropoda population formed a reverse J-shaped structure only up to 3540–3720 m asl. The maximum total biomass and carbon density were recorded in the lowest elevational range, and decreased subsequently. The primary soil nutrients under study decreased sharply along the elevational gradient. Seedling, sapling and tree distributions had a significantly positive relationship (p < 0.05) with available N, P, K, SOC, silt and clay contents and were negatively correlated (p < 0.05) with sand contents. The outcome of the study will form the basis for devising a plan for the management and conservation of J. macropoda forests. © 2022 by the authors.},
note = {2},
keywords = {},
pubstate = {published},
tppubtype = {article}
}
Salaeh, N.; Ditthakit, P.; Pinthong, S.; Hasan, M. Abul; Islam, S.; Mohammadi, B.; Linh, N. T. T.
Long-Short Term Memory Technique for Monthly Rainfall Prediction in Thale Sap Songkhla River Basin, Thailand Journal Article
In: Symmetry, vol. 14, no. 8, 2022, ISSN: 20738994, (2).
@article{2-s2.0-85137359364,
title = {Long-Short Term Memory Technique for Monthly Rainfall Prediction in Thale Sap Songkhla River Basin, Thailand},
author = { N. Salaeh and P. Ditthakit and S. Pinthong and M. Abul Hasan and S. Islam and B. Mohammadi and N.T.T. Linh},
url = {https://www.scopus.com/inward/record.uri?eid=2-s2.0-85137359364&doi=10.3390%2fsym14081599&partnerID=40&md5=c85459ae001ca9bdd572b48bf7d585c5},
doi = {10.3390/sym14081599},
issn = {20738994},
year = {2022},
date = {2022-01-01},
journal = {Symmetry},
volume = {14},
number = {8},
publisher = {MDPI},
abstract = {Rainfall is a primary factor for agricultural production, especially in a rainfed agricultural region. Its accurate prediction is therefore vital for planning and managing farmers’ plantations. Rainfall plays an important role in the symmetry of the water cycle, and many hydrological models use rainfall as one of their components. This paper aimed to investigate the applicability of six machine learning (ML) techniques (i.e.; M5 model tree: (M5); random forest: (RF); support vector regression with polynomial (SVR-poly) and RBF kernels (SVR- RBF); multilayer perceptron (MLP); and long-short-term memory (LSTM) in predicting for multiple-month ahead of monthly rainfall. The experiment was set up for two weather gauged stations located in the Thale Sap Songkhla basin. The model development was carried out by (1) selecting input variables; (2) tuning hyperparameters; (3) investigating the influence of climate variables on monthly rainfall prediction; and (4) predicting monthly rainfall with multi-step-ahead prediction. Four statistical indicators including correlation coefficient (r); mean absolute error (MAE); root mean square error (RMSE); and overall index (OI) were used to assess the model’s effectiveness. The results revealed that large-scale climate variables; particularly sea surface temperature; were significant influence variables for rainfall prediction in the tropical climate region. For projections of the Thale Sap Songkhla basin as a whole; the LSTM model provided the highest performance for both gauged stations. The developed predictive rainfall model for two rain gauged stations provided an acceptable performance: r (0.74); MAE (86.31 mm); RMSE (129.11 mm); and OI (0.70) for 1 month ahead; r (0.72); MAE (91.39 mm); RMSE (133.66 mm); and OI (0.68) for 2 months ahead; and r (0.70); MAE (94.17 mm); RMSE (137.22 mm); and OI (0.66) for 3 months ahead. © 2022 by the authors.},
note = {2},
keywords = {},
pubstate = {published},
tppubtype = {article}
}
Hossain, S. A.; Mondal, I.; ElMokh, O.; Linh, N. T. T.; Anh, D. Tran
In: Acta Geophysica, vol. 70, no. 4, pp. 1701-1715, 2022, ISSN: 18956572, (8).
@article{2-s2.0-85130725056,
title = {Assessing the multi-decadal shoreline dynamics along the Purba Medinipur-Balasore coastal stretch, India by integrating remote sensing and statistical methods},
author = { S.A. Hossain and I. Mondal and O. ElMokh and N.T.T. Linh and D. Tran Anh},
url = {https://www.scopus.com/inward/record.uri?eid=2-s2.0-85130725056&doi=10.1007%2fs11600-022-00797-5&partnerID=40&md5=52ccf7518de919296d06c1f15534f02e},
doi = {10.1007/s11600-022-00797-5},
issn = {18956572},
year = {2022},
date = {2022-01-01},
journal = {Acta Geophysica},
volume = {70},
number = {4},
pages = {1701-1715},
publisher = {Springer Science and Business Media Deutschland GmbH},
abstract = {Monitoring decadal shoreline change is essential to understand the influence of coastal processes on the coastline. The shoreline is constantly shaped by natural and anthropogenic factors, and so, it is critical to understand decadal trends. The prediction of future shoreline positions is a must for effective long-term coastal zone management. This study was conducted along a 90-km-stretch of the coastline from the mouth of the Haldi River (Purba Medinipur) in the Northeast to the Subarnarekha estuary (Balasore) in the Southwest. The primary objectives of the study were to analyze the decadal shoreline migration using the End Point Rate (EPR) method and then predict future shoreline change prediction using the Kalman Filter method. Shoreline positions were digitized after extracting the shorelines using Principal Component Analysis (PCA) from Multi-temporal (1990; 2000; 2010; and 2020) and Multisensor (Landsat TM; ETM + ; and OLI) satellite data. A total of 887 transects were cast to compute change statistics of the time series shoreline. It was observed that the average shoreline change rate was − 8.41 m/year in the periods of 1990–2000 and 2000–2010, and − 8.80 m/year from 2010 to 2020. Accretion along this coastal stretch is caused by the growth of morphological features such as sand bars, beaches, and dunes. We also found that erosion occurred from 1990 to 2000 along the coastline of Bhograi, Ramnagar-I, Ramnagar-II, a few parts of Contai-I, Khejuri-I, and the Nandigram-I coastal block. Accretion mostly occurred due to Land reclamation in the Northern portion of Bhograi, Contai-1 blocks and Nandigram- I block from 2000 to 2010 and 2010 to2020. Root mean square error (RMSE) and Regression Coefficient values were computed for the future shoreline prediction of 2031 and 2041. The calculated RMSE value of ± 4.7 m and value of 0.97 shows a good relationship between the actual and predicted coastline of 2020. This study concludes that the coastline of Purba Medinipur-Balasore experienced severe erosion and needs management action and also proves the efficiency of the Digital Shoreline Analysis System (DSAS) tool for decadal analysis and prediction of shoreline change. The findings of this study may help the coastal planners, environmentalists, and coastal managers in preparing both short-term and long-term coastal zone management plans. © 2022, The Author(s) under exclusive licence to Institute of Geophysics, Polish Academy of Sciences & Polish Academy of Sciences.},
note = {8},
keywords = {},
pubstate = {published},
tppubtype = {article}
}
Munawar, S.; Rahman, G.; Moazzam, M. F. U.; Miandad, M.; Ullah, K.; Al-Ansari, N.; Linh, N. T. T.
In: Atmosphere, vol. 13, no. 6, 2022, ISSN: 20734433, (1).
@article{2-s2.0-85132311310,
title = {Future Climate Projections Using SDSM and LARS-WG Downscaling Methods for CMIP5 GCMs over the Transboundary Jhelum River Basin of the Himalayas Region},
author = { S. Munawar and G. Rahman and M.F.U. Moazzam and M. Miandad and K. Ullah and N. Al-Ansari and N.T.T. Linh},
url = {https://www.scopus.com/inward/record.uri?eid=2-s2.0-85132311310&doi=10.3390%2fatmos13060898&partnerID=40&md5=f6e081f73164b1fbb04aab37e970b59c},
doi = {10.3390/atmos13060898},
issn = {20734433},
year = {2022},
date = {2022-01-01},
journal = {Atmosphere},
volume = {13},
number = {6},
publisher = {MDPI},
abstract = {Climate change is one of the leading issues affecting river basins due to its direct impacts on the cryosphere and hydrosphere. General circulation models (GCMs) are widely applied tools to assess climate change but the coarse spatial resolution of GCMs limit their direct application for local studies. This study selected five CMIP5 GCMs (CCSM4; HadCM3; GFDL-CM3; MRI-CGCM3 and CanESM2) for performance evaluation ranked by Nash–Sutcliffe coefficient (NSE) and Kling–Gupta Efficiency (KGE). CCSM4 and HadCM3 large-scale predictors were favored based on ranks (0.71 and 0.68; respectively) for statistical downscaling techniques to downscale the climatic indicators Tmax, Tmin and precipitation. The performance of two downscaling techniques, Statistical Downscaling Methods (SDSM) and Long Ashton Research Station Weather Generator (LARS-WG), were examined using the Mean Absolute Error (MAE), Root Mean Square Error (RMSE), bias, NSE and KGE with weights (Wi) for the validation period. The results of statistical measures proved SDSM more efficient (0.67) in comparison to the LARS-WG (0.51) for the validation time for the Jhelum River basin. The findings revealed that the SDSM simulation for Tmax and Tmin are more comparable to the reference data for the validation period except simulation of extreme events by precipitation. The 21st century climatic projections exhibited a significant rise in Tmax (2.37–4.66◦C), Tmin (2.47–4.52◦C) and precipitation (7.4–11.54%) for RCP-4.5 and RCP-8.5, respectively. Overall, the results depicted that winter and pre-monsoon seasons were potentially most affected in terms of warming and precipitation, which has the potential to alter the cryosphere and runoff of the Jhelum River basin. © 2022 by the authors. Licensee MDPI, Basel, Switzerland.},
note = {1},
keywords = {},
pubstate = {published},
tppubtype = {article}
}
Sarfraz, Y.; Basharat, M.; Riaz, M. T.; Akram, M. S.; Xu, Cho.; Ahmed, K. S.; Shahzad, A.; Al-Ansari, N.; Linh, N. T. T.
Application of statistical and machine learning techniques for landslide susceptibility mapping in the Himalayan road corridors Journal Article
In: Open Geosciences, vol. 14, no. 1, pp. 1606-1635, 2022, ISSN: 23915447.
@article{2-s2.0-85145606589,
title = {Application of statistical and machine learning techniques for landslide susceptibility mapping in the Himalayan road corridors},
author = { Y. Sarfraz and M. Basharat and M.T. Riaz and M.S. Akram and Cho. Xu and K.S. Ahmed and A. Shahzad and N. Al-Ansari and N.T.T. Linh},
url = {https://www.scopus.com/inward/record.uri?eid=2-s2.0-85145606589&doi=10.1515%2fgeo-2022-0424&partnerID=40&md5=f461144014dec179041578fe0ffa000a},
doi = {10.1515/geo-2022-0424},
issn = {23915447},
year = {2022},
date = {2022-01-01},
journal = {Open Geosciences},
volume = {14},
number = {1},
pages = {1606-1635},
publisher = {De Gruyter Open Ltd},
abstract = {Landslides are frequent geological hazards, mainly in the rainy season along road corridors worldwide. In the present study, we have comparatively analyzed landslide susceptibility by employing integrated geospatial approaches, i.e., data-driven, knowledge-driven, andmachine learning (ML), along themain road corridors of the Muzaffarabad district. The landslide inventory of three road corridors is developed to evaluate landslide susceptibility, and eleven landslide causative factors (LCFs) were analyzed. After statistical significance analysis, these eleven LCFs generated susceptibility models using WoE, AHP, LR, and RF. Distance from roads, landcover, lithological units, and slopes are considered more influential LCFs. The performancematrix of different LSMs is evaluated through the area under the curve (AUC-ROC), overall accuracy, Kappa index, F1 score, Mean Absolute Error, and Root Mean Square Error. The AUC-ROC for WoE, AHP, LR, and RF techniques along Neelumroad is 0.86, 0.82, 0.91, and 0.97, respectively, along Jhelum Valley road is 0.83, 0.81, 0.93, and 0.95, respectively, while along Kohala road is 0.89, 0.88, 0.89, and 0.92, respectively. The produced LSMs through ML (i.e.; RF and LR) showed better prediction accuracies than WoE and AHP along these three road corridors. The LSMs are categorized into very high, high, moderate, and low susceptible zones along these roads. The LSM generated through hybrid models can facilitate the concerned local agencies to implement landslide mitigation policies for the landslideprone zones along road corridors. © 2022 the author(s).},
keywords = {},
pubstate = {published},
tppubtype = {article}
}
Pinthong, S.; Ditthakit, P.; Salaeh, N.; Hasan, M. Abul; Son, C. T.; Linh, N. T. T.; Islam, S.; Yadav, K. K.
In: Environmental Science and Pollution Research, 2022, ISSN: 09441344, (2).
@article{2-s2.0-85139148285,
title = {Imputation of missing monthly rainfall data using machine learning and spatial interpolation approaches in Thale Sap Songkhla River Basin, Thailand},
author = { S. Pinthong and P. Ditthakit and N. Salaeh and M. Abul Hasan and C.T. Son and N.T.T. Linh and S. Islam and K.K. Yadav},
url = {https://www.scopus.com/inward/record.uri?eid=2-s2.0-85139148285&doi=10.1007%2fs11356-022-23022-8&partnerID=40&md5=c23ae9f55551f88266973d7f9c3b24aa},
doi = {10.1007/s11356-022-23022-8},
issn = {09441344},
year = {2022},
date = {2022-01-01},
journal = {Environmental Science and Pollution Research},
publisher = {Springer Science and Business Media Deutschland GmbH},
abstract = {Missing rainfall data has been a prevalent issue and primarily interested in hydrology and meteorology. This research aimed to examine the capability of machine learning (ML) and spatial interpolation (SI) methods to estimate missing monthly rainfall data. Six ML algorithms (i.e. multiple linear regression (MLR); M5 model tree (M5); random forest (RF); support vector regression (SVR); multilayer perceptron (MLP); genetic programming (GP)) and four SI methods (i.e. arithmetic average (AA); inverse distance weighting (IDW); correlation coefficient weighted (CCW); normal ratio (NR)) were investigated and compared in their performance. The twelve rainfall stations, located in the Thale Sap Songkhla river basin and nearby basins, were considered as a study case. Tuning hyper-parameters for each ML method was conducted to get the most suitable model for the data sets considered. Three performance criteria matrices (i.e. NSE; OI; and r) were chosen, and the sum of those three performance criteria matrices was introduced for methods’ performance comparison. The experimental results pointed out that selecting neighbouring stations were essential when applying SI methods, but not for the ML method. The overall performance showed ML better imputed missing monthly rainfall than SI due to overcoming spatial constraints. GP provided the highest performance by giving NSE = 0.825},
note = {2},
keywords = {},
pubstate = {published},
tppubtype = {article}
}
El-Magd, S. A. Abu; Ahmed, H.; Pham, Q. B.; Linh, N. T. T.; Anh, D. Tran; Elkhrachy, I.; Masoud, A. M.
In: Water (Switzerland), vol. 14, no. 24, 2022, ISSN: 20734441, (5).
@article{2-s2.0-85144634572,
title = {Possible Factors Driving Groundwater Quality and Its Vulnerability to Land Use, Floods, and Droughts Using Hydrochemical Analysis and GIS Approaches},
author = { S.A. Abu El-Magd and H. Ahmed and Q.B. Pham and N.T.T. Linh and D. Tran Anh and I. Elkhrachy and A.M. Masoud},
url = {https://www.scopus.com/inward/record.uri?eid=2-s2.0-85144634572&doi=10.3390%2fw14244073&partnerID=40&md5=c9e0130a5eef7597592bc93710383c5f},
doi = {10.3390/w14244073},
issn = {20734441},
year = {2022},
date = {2022-01-01},
journal = {Water (Switzerland)},
volume = {14},
number = {24},
publisher = {MDPI},
abstract = {Land use and climate change always induce significant changes in various parameters of the hydrologic cycle (e.g.; surface runoff; infiltration; evapotranspiration). The Wadi El-Assiuti downstream area in the Eastern Desert of Egypt is one of the most promising areas for development that is suffering from insufficient water availability and inadequate water quality for different purposes. The main goal of this research is to evaluate the changes in groundwater quality, land use, and climate in association with geology and flooding during three periods within the years 1997–2019 in the downstream portion of Wadi El-Assiuti in the Eastern Desert of Egypt, using spatiotemporal variation associated with groundwater hydrochemical analysis and GIS techniques. About 133 groundwater samples were collected to examine groundwater quality changes over time. Different groundwater quality indices were calculated, and the results show that TDS levels of groundwater in the study area ranged between 1080–2780 mg/L, 672–4564 mg/L, and 811–6084 mg/L, while SAR levels varied within 6.15–15.34, 1.83–28.87, and 1.43–30.57 for the years 1997, 2007, and 2019, respectively. Both RSBC and SSP values exhibited significantly increasing trends over time. KR values were within 1.36–4.06 in 1997, 0.58–14.09 in 2007, and 0.35–14.92 in 2019; MAR values were within 6.9–45.2 in 1997, 20.79–71.5 in 2007, and 17.71–75.81 in 2019; and PI values were within 60.16–83 in 1997, 45.56–101.03 in 2007, and 42.51–148.88 in 2019. Across the entire study area, ongoing land use changes increased from 1.1% in 1997 to 4.1% in 2019. Findings pointed to the significant contribution of the deep Nubian Sandstone Aquifer to the groundwater aquifer at Wadi El-Assiuti through fractures and deep faults. Given the climatic conditions from 1997–2019, these changes may have affected water quality in shallow aquifers, especially with increasing evaporation. Realizing the spatiotemporal variation of the aquifer recharge system, land use development, and climate change clearly would help in water resource management. This study revealed that flooding events, deep-seated geologic structures, and land use development associated with human activities have the highest impact on groundwater quality. © 2022 by the authors.},
note = {5},
keywords = {},
pubstate = {published},
tppubtype = {article}
}
Weekaew, J.; Ditthakit, P.; Pham, Q. B.; Kittiphattanabawon, N.; Linh, N. T. T.
Comparative Study of Coupling Models of Feature Selection Methods and Machine Learning Techniques for Predicting Monthly Reservoir Inflow Journal Article
In: Water (Switzerland), vol. 14, no. 24, 2022, ISSN: 20734441, (2).
@article{2-s2.0-85144624873,
title = {Comparative Study of Coupling Models of Feature Selection Methods and Machine Learning Techniques for Predicting Monthly Reservoir Inflow},
author = { J. Weekaew and P. Ditthakit and Q.B. Pham and N. Kittiphattanabawon and N.T.T. Linh},
url = {https://www.scopus.com/inward/record.uri?eid=2-s2.0-85144624873&doi=10.3390%2fw14244029&partnerID=40&md5=1e8b8f082bf3976792326d57d88a40c5},
doi = {10.3390/w14244029},
issn = {20734441},
year = {2022},
date = {2022-01-01},
journal = {Water (Switzerland)},
volume = {14},
number = {24},
publisher = {MDPI},
abstract = {Effective reservoir operation under the effects of climate change is immensely challenging. The accuracy of reservoir inflow forecasting is one of the essential factors supporting reservoir operations. This study aimed to investigate coupling models of feature selection (FS) and machine learning (ML) algorithms to predict the monthly reservoir inflow. The study was carried out using data from the Huai Nam Sai reservoir in southern Thailand. Eighteen years of monthly recorded data (i.e.; reservoir inflow; reservoir storage; rainfall; and regional climate indices) with up to a 12-month time lag were utilized. Three ML techniques, i.e., multiple linear regression (MLR), support vector regression (SVR), and artificial neural network (ANN)were compared in their capabilities. In addition, two FS techniques, i.e., genetic algorithm (GA) and backward elimination (BE) methods, were studied with four predictable time intervals, consisting of 3, 6, 9, and 12 months in advance. Ten-fold cross-validation was used for model evaluation. Study results revealed that FS methods (i.e.; GA and BE) Could improve the performance of SVR and ANN for predicting monthly reservoir inflow forecasting, but they have no effects on MLR. Different developed forecasting models were suitable for different reservoir inflow forecasting time-step-ahead. BE-ANN provided the best performance for three-time-ahead (T + 3) and nine-time-ahead (T + 9) by giving an OI of 0.9885 and 0.8818, NSE of 0.9546 and 0.9815, RMSE of 1.3155 and 1.2172 MCM/month, MAE of 0.9568 and 0.9644 MCM/month, and r of 0.9796 and 0.9804, respectively. The GA-ANN model showed the highest prediction accuracy for six-time-ahead (T + 6), with an OI of 0.8997, NSE of 0.9407, RMSE of 2.1699 MCM/month, MAE of 1.7549 MCM/month, and r of 0.9759. The ANN model showed the best prediction accuracy for twelve-time-ahead (T + 12), with an OI of 0.9515, NSE of 0.9835, RMSE of 1.1613 MCM/month, MAE of 0.9273 MCM/month, and r of 0.9835. © 2022 by the authors.},
note = {2},
keywords = {},
pubstate = {published},
tppubtype = {article}
}
Ogunrinde, A. T.; Oguntunde, P. G.; Akinwumiju, A. S.; Fasinmirin, J. T.; Olasehinde, D. A.; Pham, Q. B.; Linh, N. T. T.; Anh, D. Tran
Impact of Climate Change and Drought Attributes in Nigeria Journal Article
In: Atmosphere, vol. 13, no. 11, 2022, ISSN: 20734433.
@article{2-s2.0-85141713255,
title = {Impact of Climate Change and Drought Attributes in Nigeria},
author = { A.T. Ogunrinde and P.G. Oguntunde and A.S. Akinwumiju and J.T. Fasinmirin and D.A. Olasehinde and Q.B. Pham and N.T.T. Linh and D. Tran Anh},
url = {https://www.scopus.com/inward/record.uri?eid=2-s2.0-85141713255&doi=10.3390%2fatmos13111874&partnerID=40&md5=4110d0c2a978c97ba6e27de30594bede},
doi = {10.3390/atmos13111874},
issn = {20734433},
year = {2022},
date = {2022-01-01},
journal = {Atmosphere},
volume = {13},
number = {11},
publisher = {MDPI},
abstract = {Data from historical observatories and future simulations were analyzed using the representative concentration pathway (RCP) 8.5 scenario, which covered the period from 1951 to 2100. In order to characterize the drought, three widely used drought indicators were used: the standardized precipitation index (SPI), the reconnaissance drought index (RDI), and the standardized precipitation and evapotranspiration index (SPEI). The ensemble of the seven (7) GCMs that used RCA-4 was able to capture several useful characteristics of Nigeria’s historical climatology. Future climates were forecasted to be wetter than previous periods during the study period based on the output of drought characteristics as determined by SPI. SPEI and RDI predicted drier weather, in contrast. SPEI and RDI’s predictions must have been based on the effect of rising temperatures brought on by global warming as depicted by RCP 8.5, which would then have an impact on the rate of evapotranspiration. According to drought studies using the RCP 8.5 scenario, rising temperatures will probably cause more severe/extreme droughts to occur more frequently. SPEI drought frequency changes in Nigeria often range from 0.75 (2031–2060) to 1.80 (2071–2100) month/year, whereas RDI changes typically range from 0.30 (2031–2060) to 0.60 (2071–2100) month/year. The frequency of drought incidence has recently increased and is now harder to forecast. Since the Sendai Framework for Disaster Risk Reduction 2015–2030 (SFDRR) and the Sustainable Development Goals (SDGs) have few more years left to be completed, drastic efforts must be made to create climate-resilient systems that can tackle the effects that climate change may have on the water resources and agricultural sectors. © 2022 by the authors.},
keywords = {},
pubstate = {published},
tppubtype = {article}
}
Ifkirne, M.; Bouhi, H. El; Acharki, S.; Pham, Q. B.; Farah, A.; Linh, N. T. T.
Multi-Criteria GIS-Based Analysis for Mapping Suitable Sites for Onshore Wind Farms in Southeast France Journal Article
In: Land, vol. 11, no. 10, 2022, ISSN: 2073445X, (1).
@article{2-s2.0-85140715523,
title = {Multi-Criteria GIS-Based Analysis for Mapping Suitable Sites for Onshore Wind Farms in Southeast France},
author = { M. Ifkirne and H. El Bouhi and S. Acharki and Q.B. Pham and A. Farah and N.T.T. Linh},
url = {https://www.scopus.com/inward/record.uri?eid=2-s2.0-85140715523&doi=10.3390%2fland11101839&partnerID=40&md5=82019da7bcadc5eb4aa1b60e50b42df2},
doi = {10.3390/land11101839},
issn = {2073445X},
year = {2022},
date = {2022-01-01},
journal = {Land},
volume = {11},
number = {10},
publisher = {MDPI},
abstract = {Wind energy is critical to traditional energy sources replacement in France and throughout the world. Wind energy generation in France is quite unevenly spread across the country. Despite its considerable wind potential, the research region is among the least productive. The region is a very complicated location where socio-environmental, technological, and topographical restrictions intersect, which is why energy production planning studies in this area have been delayed. In this research, the methodology used for identifying appropriate sites for future wind farms in this region combines GIS with MCDA approaches such as AHP. Six determining factors are selected: the average wind speed, which has a weight of 38%; the protected areas, which have a relative weight of 26%; the distance to electrical substations and road networks, both of which have a significant influence on relative weights of 13%; and finally, the slope and elevation, which have weights of 5% and 3%, respectively. Only one alternative was investigated (suitable and unsuitable). The spatial database was generated using ArcGIS and QGIS software; the AHP was computed using Excel; and several treatments, such as raster data categorization and weighted overlay, were automated using the Python programming language. The regions identified for wind turbines installation are defined by a total of 962,612 pixels, which cover a total of 651 km2 and represent around 6.98% of the research area. The theoretical wind potential calculation results suggest that for at least one site with an area bigger than 400 ha, the energy output ranges between 182.60 and 280.20 MW. The planned sites appear to be suitable; each site can support an average installed capacity of 45 MW. This energy benefit will fulfill the region’s population’s transportation, heating, and electrical demands. © 2022 by the authors.},
note = {1},
keywords = {},
pubstate = {published},
tppubtype = {article}
}
Thongkao, S.; Ditthakit, P.; Pinthong, S.; Salaeh, N.; Elkhrachy, I.; Linh, N. T. T.; Pham, Q. B.
Estimating FAO Blaney-Criddle b-Factor Using Soft Computing Models Journal Article
In: Atmosphere, vol. 13, no. 10, 2022, ISSN: 20734433, (2).
@article{2-s2.0-85140483566,
title = {Estimating FAO Blaney-Criddle b-Factor Using Soft Computing Models},
author = { S. Thongkao and P. Ditthakit and S. Pinthong and N. Salaeh and I. Elkhrachy and N.T.T. Linh and Q.B. Pham},
url = {https://www.scopus.com/inward/record.uri?eid=2-s2.0-85140483566&doi=10.3390%2fatmos13101536&partnerID=40&md5=2560e37aa9596a6a96d2ad7ef74c5649},
doi = {10.3390/atmos13101536},
issn = {20734433},
year = {2022},
date = {2022-01-01},
journal = {Atmosphere},
volume = {13},
number = {10},
publisher = {MDPI},
abstract = {FAO Blaney-Criddle has been generally an accepted method for estimating reference crop evapotranspiration. In this regard, it is inevitable to estimate the b-factor provided by the Food and Agriculture Organization (FAO) of the United Nations Irrigation and Drainage Paper number 24. In this study, five soft computing methods, namely random forest (RF), M5 model tree (M5), support vector regression with the polynomial function (SVR-poly), support vector regression with radial basis function kernel (SVR-rbf), and random tree (RT), were adapted to estimate the b-factor. And Their performances were also compared. The suitable hyper-parameters for each soft computing method were investigated. Five statistical indices were deployed to evaluate their performance, i.e., the coefficient of determination (r2), the mean absolute relative error (MARE), the maximum absolute relative error (MXARE), the standard deviation of the absolute relative error (DEV), and the number of samples with an error greater than 2% (NE > 2%). Findings reveal that SVR-rbf gave the highest performance among five soft computing models, followed by the M5, RF, SVR-poly, and RT. The M5 also derived a new explicit equation for b estimation. SVR-rbf provided a bit lower efficacy than the radial basis function network but outperformed the regression equations. Models’ Applicability for estimating monthly reference evapotranspiration (ETo) was demonstrated. © 2022 by the authors.},
note = {2},
keywords = {},
pubstate = {published},
tppubtype = {article}
}
Hussain, A.; Rahman, K. U.; Shahid, M. N.; Haider, S.; Pham, Q. B.; Linh, N. T. T.; Sammen, S. S.
In: Environment, Development and Sustainability, vol. 24, no. 9, pp. 10852-10875, 2022, ISSN: 1387585X.
@article{2-s2.0-85116744296,
title = {Investigating feasible sites for multi-purpose small dams in Swat District of Khyber Pakhtunkhwa Province, Pakistan: socioeconomic and environmental considerations},
author = { A. Hussain and K.U. Rahman and M.N. Shahid and S. Haider and Q.B. Pham and N.T.T. Linh and S.S. Sammen},
url = {https://www.scopus.com/inward/record.uri?eid=2-s2.0-85116744296&doi=10.1007%2fs10668-021-01886-z&partnerID=40&md5=281eee1d44c47d0c8accd863de4ae98b},
doi = {10.1007/s10668-021-01886-z},
issn = {1387585X},
year = {2022},
date = {2022-01-01},
journal = {Environment, Development and Sustainability},
volume = {24},
number = {9},
pages = {10852-10875},
publisher = {Springer Science and Business Media B.V.},
abstract = {The low water storage capacity caused water crisis in Pakistan; therefore, the country needs both small- and large-scale reservoirs to store surplus water resources. The construction of large dams in Pakistan could not be materialized due to financial and political constraints. However, the construction of multi-purpose small dams is the next best option to store water. To this end, there is a need to identify the best feasible sites in the country. The selection of feasible sites for multi-purpose dams must take into account multiple criteria, including engineering, socioeconomic and environmental. The current study utilizes the coupled Remote Sensing and Geographical Information System techniques to identify the feasible sites for multi-purpose small dams, considering the socioeconomic and environmental criteria in addition to the established engineering criteria in district Swat, Khyber Pakhtunkhwa. The suitability map considered nine engineering criteria, including rainfall distribution, slope, land use, curve number, runoff depth, soil, alluvial depth, closeness to streams, and drainage density, using the weights calculated from priority indices. The suitability map is divided into four classes, i.e., excellent, good, moderate, and unsuitable with the excellent and good classes area of 66.78 km2, and 195.75 km2. Twenty sites (based on accessibility and closeness to Swat River) from each class are selected that are situated in Kalam, Babozai, Bahrain, Madyan, Khwazakhela, Matta, Kabal, and Barikot areas of district Swat and evaluated using socioeconomic and environmental criteria, i.e., community well and no displacement cost, management ownership–private or public, biodiversity protection services, instrumental in groundwater recharge for the community, electricity generation for the local community, low maintenance cost, flood friendly, appropriate distribution of water resources, political well, and irrigation and drinking water potential. The top priority for these areas is electricity generation, flood protection, irrigation and drinking water capability, sustainable operation, low maintenance cost and political well. The current study demonstrated that socioeconomic and environmental criteria augment the engineering approach in identifying the best feasible site for multi-purpose small dams. These sites would not only store the water but would also provide important services (electricity generation; irrigation water; etc.) to the local community and economy. © 2021, The Author(s), under exclusive licence to Springer Nature B.V.},
keywords = {},
pubstate = {published},
tppubtype = {article}
}
Parvin, F.; Ali, S. A.; Calka, B.; Bielecka, E.; Linh, N. T. T.; Pham, Q. B.
Urban flood vulnerability assessment in a densely urbanized city using multi-factor analysis and machine learning algorithms Journal Article
In: Theoretical and Applied Climatology, vol. 149, no. 1-2, pp. 639-659, 2022, ISSN: 0177798X, (4).
@article{2-s2.0-85129549555,
title = {Urban flood vulnerability assessment in a densely urbanized city using multi-factor analysis and machine learning algorithms},
author = { F. Parvin and S.A. Ali and B. Calka and E. Bielecka and N.T.T. Linh and Q.B. Pham},
url = {https://www.scopus.com/inward/record.uri?eid=2-s2.0-85129549555&doi=10.1007%2fs00704-022-04068-7&partnerID=40&md5=3f69367a1a43cdc2f07dd05cade624ff},
doi = {10.1007/s00704-022-04068-7},
issn = {0177798X},
year = {2022},
date = {2022-01-01},
journal = {Theoretical and Applied Climatology},
volume = {149},
number = {1-2},
pages = {639-659},
publisher = {Springer},
abstract = {Flood is considered as the most devastating natural hazards that cause the death of many lives worldwide. The present study aimed to predict flood vulnerability for Warsaw, Poland, using three machine learning models, such as the Bayesian logistic regression (BLR), the artificial neural networks (ANN), and the deep learning neural networks (DLNNs). The perfomance of these three methods was assessed in order to select the best method for flood vulnerability mapping in densely urbanized city. Thus, initially, thirteen flood predictors were evaluated using the information gain ratio (IGR), and eight most important predictors were considered from model training and testing. The performance of the applied models and accuracy of the result was evaluated through the area under the curve (AUC) and statistical measures. By using the testing dataset, the result reveals that DLNN (AUC = 0.877) is the more performant model in comparison to ANN (AUC = 0.851) and BLR (AUC = 0.697). However, the BLR model has the lowest predictive capability. The results of the present study could be effectively used for the urban flood management strategies. © 2022, The Author(s), under exclusive licence to Springer-Verlag GmbH Austria, part of Springer Nature.},
note = {4},
keywords = {},
pubstate = {published},
tppubtype = {article}
}
Pal, S. C.; Chakrabortty, R.; Saha, A.; Bozchaloei, S. K.; Pham, Q. B.; Linh, N. T. T.; Anh, D. Tran; Janizadeh, S.; Ahmadi, K.
Evaluation of debris flow and landslide hazards using ensemble framework of Bayesian- and tree-based models Journal Article
In: Bulletin of Engineering Geology and the Environment, vol. 81, no. 1, 2022, ISSN: 14359529, (15).
@article{2-s2.0-85122310778,
title = {Evaluation of debris flow and landslide hazards using ensemble framework of Bayesian- and tree-based models},
author = { S.C. Pal and R. Chakrabortty and A. Saha and S.K. Bozchaloei and Q.B. Pham and N.T.T. Linh and D. Tran Anh and S. Janizadeh and K. Ahmadi},
url = {https://www.scopus.com/inward/record.uri?eid=2-s2.0-85122310778&doi=10.1007%2fs10064-021-02546-2&partnerID=40&md5=28e590545f4407e570d937b8e313d318},
doi = {10.1007/s10064-021-02546-2},
issn = {14359529},
year = {2022},
date = {2022-01-01},
journal = {Bulletin of Engineering Geology and the Environment},
volume = {81},
number = {1},
publisher = {Springer Science and Business Media Deutschland GmbH},
abstract = {The modeling and prediction of land movement susceptibility hazards, i.e., debris flow, landslide, and rock fall, can assist in controlling and preventing a variety of societal and environmental damages. The purpose of this study was to develop a land movement susceptibility hazard model of debris flow, landslide, and multiple land movement, i.e., combination of debris flow and landslide in the Saveh city of Markazi Province, Iran, using an ensemble of Bayesian generalized linear model (BGLM), sparse partial least squares (SPLS), boosted tree (BT), and random forest (RF) algorithms. For this purpose, 167 debris flow points, 261 landslide points, and 257 multiple (debris flow and landslide) points were identified based on field visits and available information, and 15 suitable conditioning factors were prepared as independent variables for this study. The accuracy and efficiency of the models were assessed using the receiver operating characteristic (ROC) and other statistical indices. The variable importance result indicates that slope is the most important factor in debris flow (25.53), landslide (31.39), and multiple hazard (41.90) occurrences. The accuracy assessment results in the validation phase revealed that the RF is the most optimal among the applied algorithms, with area under the curve (AUC) values of 0.90, 0.94, and 0.89 for debris flow, landslide, and multiple (D + L) hazard modeling. The findings of this study indicated that the use of a Bayesian and tree-based ensemble model in preparing a land movement-related disaster map could be useful among policymakers and land use planners for sustainable land use management and practices. © 2022, Springer-Verlag GmbH Germany, part of Springer Nature.},
note = {15},
keywords = {},
pubstate = {published},
tppubtype = {article}
}
Chen, Y.; Chen, W.; Janizadeh, S.; Bhunia, G. S.; Bera, A.; Pham, Q. B.; Linh, N. T. T.; Balogun, A. L.; Wang, X.
In: Geocarto International, vol. 37, no. 16, pp. 4628-4654, 2022, ISSN: 10106049, (24).
@article{2-s2.0-85102922157,
title = {Deep learning and boosting framework for piping erosion susceptibility modeling: spatial evaluation of agricultural areas in the semi-arid region},
author = { Y. Chen and W. Chen and S. Janizadeh and G.S. Bhunia and A. Bera and Q.B. Pham and N.T.T. Linh and A.L. Balogun and X. Wang},
url = {https://www.scopus.com/inward/record.uri?eid=2-s2.0-85102922157&doi=10.1080%2f10106049.2021.1892212&partnerID=40&md5=c07a8225880c124b52f4ed8fb0d9a916},
doi = {10.1080/10106049.2021.1892212},
issn = {10106049},
year = {2022},
date = {2022-01-01},
journal = {Geocarto International},
volume = {37},
number = {16},
pages = {4628-4654},
publisher = {Taylor and Francis Ltd.},
abstract = {Piping erosion is one of the water erosions that cause significant changes in the landscape, leading to environmental degradation. To prevent losses resulting from tube growth and enable sustainable development, developing high-precision predictive algorithms for piping erosion is essential. Boosting is a classic algorithm that has been successfully applied to diverse computer vision tasks. Therefore, this work investigated the predictive performance of the Boosted Linear Model (BLM), Boosted Regression Tree (BRT), Boosted Generalized Linear Model (Boost GLM), and Deep Boosting models for piping erosion susceptibility mapping in Zarandieh Watershed located in the Markazi province of Iran. A piping inventory map including 152 piping erosion locations was prepared for algorithm training and testing. 18 initial predisposing factors (altitude; slope; plan curvature; profile curvature; distance from river; drainage density; distance from road; rainfall; land use; soil type; bulk density; CEC; pH; clay; silt; sand; topographical position index (TPI); topographic wetness index (TWI)) was derived from multiple remote sensing (RS) sources to determine the piping erosion prone areas. The most significant predisposing factors were selected using multi-collinearity analysis which indicates linear correlations between predisposing factors. Finally, the results were evaluated for Sensitivity, Specificity, Positive predictive values (PPV) and Negative predictive value (NPV), and Receiver Operation characteristic (ROC) curve. The best Sensitivity (0.80), Specificity (0.84), PPV (0.85), NPV (0.79), and ROC (0.93), were obtained by Deep Boosting model. The results of the piping erosion susceptibility study in agricultural land use showed that 41% of agricultural lands are very sensitive to piping erosion. This outcome will enable natural resource managers and local planners to assess and take effective decisions to minimize damages to agricultural land use by accurately identifying the most vulnerable areas. Hence, this research proved Deep Boosting model’s ability for piping erosion susceptibility mapping in comparison to other popular methods such as BLM, BRT, and Boost GLM. © 2021 Informa UK Limited, trading as Taylor & Francis Group.},
note = {24},
keywords = {},
pubstate = {published},
tppubtype = {article}
}
Yariyan, P.; Avand, M.; Omidvar, E.; Pham, Q. B.; Linh, N. T. T.; Tiefenbacher, J. P.
Optimization of statistical and machine learning hybrid models for groundwater potential mapping Journal Article
In: Geocarto International, vol. 37, no. 13, pp. 3877-3911, 2022, ISSN: 10106049, (12).
@article{2-s2.0-85100945647,
title = {Optimization of statistical and machine learning hybrid models for groundwater potential mapping},
author = { P. Yariyan and M. Avand and E. Omidvar and Q.B. Pham and N.T.T. Linh and J.P. Tiefenbacher},
url = {https://www.scopus.com/inward/record.uri?eid=2-s2.0-85100945647&doi=10.1080%2f10106049.2020.1870164&partnerID=40&md5=26f78eae2271160a277d5f453109d6aa},
doi = {10.1080/10106049.2020.1870164},
issn = {10106049},
year = {2022},
date = {2022-01-01},
journal = {Geocarto International},
volume = {37},
number = {13},
pages = {3877-3911},
publisher = {Taylor and Francis Ltd.},
abstract = {Determining areas of high groundwater potential is important for exploitation, management, and protection of water resources. This study assesses the spatial distribution of groundwater potential in the Zarrinehroud watershed of Kurdistan Province, Iran using combinations of five statistical and machine learning algorithms–frequency ratio (FR), radial basis function (RBF), index of entropy (IOE), evidential belief function (EBF) and fuzzy art map (FAM). To accomplish this, 1448 well locations in the study area were randomly divided into two data sets for training (70%= 1013 locations) and validation (30%= 435 locations) based on the holdout method. Fourteen factors that can affect the presence or absence of groundwater were identified, measured, and mapped using ArcGIS and SAGA-GIS software. The models were used to predict the locations of groundwater based on suitable combinations of the conditioning factors to produce groundwater potential maps. The probability of groundwater at any location was classified as low, moderate, high, or very high based on natural breaks in the data spectrum. The model predictions were tested for validity and their success was determined using receiver operating characteristic (ROC) curves, standard errors (SE), positive predictive value (PPV), negative predictive value (NPV), sensitivity (SST), specificity (SPF) and accuracy (ACC), and the Friedman test. The performance assessments of groundwater potential predictions using the area under the curve (AUC) and accuracy (ACC) showed that the FR-RBF model had very good performance (AUC= 0.889; ACC= 87.51). FR-FAM (AUC= 0.869; ACC= 84.67), EBF-FAM (AUC= 0.864; ACC= 84.42), EBF-RBF (AUC= 0.854; ACC= 83.94), FR-IOE (AUC= 0.836; ACC= 83.62), and EBF-IOE (AUC= 0.833; ACC= 80.42) also had acceptable performance. The results of the Friedman test also show that there are significant differences between the models and the highest mean rank was generated by the FR-FAM model (3.642). Therefore, the hybrid models can be used to increase the accuracy of groundwater-prediction models in the study region and perhaps in similar settings. Highlights The groundwater potential was studied in the area of Zarrinehroud watershed A combination of methods including FR, RBF, IOE, EBF and FAM Very high and high groundwater potential areas were located in the northern half The development of hybrid models can increase the accuracy of the results. © 2021 Informa UK Limited, trading as Taylor & Francis Group.},
note = {12},
keywords = {},
pubstate = {published},
tppubtype = {article}
}
Hassangavyar, M. B.; Damaneh, H. E.; Pham, Q. B.; Linh, N. T. T.; Tiefenbacher, J. P.; Bach, Q. V.
Evaluation of re-sampling methods on performance of machine learning models to predict landslide susceptibility Journal Article
In: Geocarto International, vol. 37, no. 10, pp. 2772-2794, 2022, ISSN: 10106049, (8).
@article{2-s2.0-85097937024,
title = {Evaluation of re-sampling methods on performance of machine learning models to predict landslide susceptibility},
author = { M.B. Hassangavyar and H.E. Damaneh and Q.B. Pham and N.T.T. Linh and J.P. Tiefenbacher and Q.V. Bach},
url = {https://www.scopus.com/inward/record.uri?eid=2-s2.0-85097937024&doi=10.1080%2f10106049.2020.1837257&partnerID=40&md5=1ff2e4ea6eb5b7fd219fcfba5d788628},
doi = {10.1080/10106049.2020.1837257},
issn = {10106049},
year = {2022},
date = {2022-01-01},
journal = {Geocarto International},
volume = {37},
number = {10},
pages = {2772-2794},
publisher = {Taylor and Francis Ltd.},
abstract = {This study tests the applicability of three resampling methods (i.e. bootstrapping; random-subsampling and cross-validation) for enhancing the performance of eight machine-learning models: boosted regression trees, flexible discriminant analysis, random forests, mixture discriminate analysis, multivariate adaptive regression splines, classification and regression trees, support vector machines and generalized linear models, compared to the use of the original data. The results of models were evaluated using correlation (COR), area under curve (AUC), true skill statistic (TSS), receiver-operating characteristic and the probability of detection (POD). The evaluation showed that the bootstrapping technique improved the performance of all models. The Bootstrapping-random forest (with COR = 0.75; AUC = 0.92; TSS = 0.80 and POD = 0.98) proved to be the best model for landslide prediction. Among the 18 contributing factors, distance from fault, curvature and precipitation were the most influential in all 32 models.Highlights Hazard prediction of landslide by the 8 machine-learning (ML) models. Multiple morphometric, climatic, geologic, vegetation and human factors were used. Tests the applicability of three resampling methods. The performance of the ML models and coupling models were assessed. © 2020 Informa UK Limited, trading as Taylor & Francis Group.},
note = {8},
keywords = {},
pubstate = {published},
tppubtype = {article}
}
2021
Dehghanisanij, H.; Emami, S.; Achite, M.; Linh, N. T. T.; Pham, Q. B.
Estimating yield and water productivity of tomato using a novel hybrid approach Journal Article
In: Water (Switzerland), vol. 13, no. 24, 2021, ISSN: 20734441, (2).
@article{2-s2.0-85121365223,
title = {Estimating yield and water productivity of tomato using a novel hybrid approach},
author = { H. Dehghanisanij and S. Emami and M. Achite and N.T.T. Linh and Q.B. Pham},
url = {https://www.scopus.com/inward/record.uri?eid=2-s2.0-85121365223&doi=10.3390%2fw13243615&partnerID=40&md5=29427366664f415cbe3926ef79bd124a},
doi = {10.3390/w13243615},
issn = {20734441},
year = {2021},
date = {2021-01-01},
journal = {Water (Switzerland)},
volume = {13},
number = {24},
publisher = {MDPI},
abstract = {Water productivity (WP) of crops is affected by water–fertilizer management in interaction with climatic factors. This study aimed to evaluate the efficiency of a hybrid method of season optimization algorithm (SO) and support vector regression (SVR) in estimating the yield and WP of tomato crops based on climatic factors, irrigation–fertilizer under the drip irrigation, and plastic mulch. To approve the proposed method, 160 field data including water consumption during the growing season, fertilizers, climatic variables, and crop variety were applied. Two types of treatments, namely drip irrigation (DI) and drip irrigation with plastic mulch (PMDI), were considered. Seven different input combinations were used to estimate yield and WP. R2, RMSE, NSE, SI, and σ criteria were utilized to assess the proposed hybrid method. A good agreement was presented between the observed (field monitoring data) and estimated (calculated with SO–SVR method) values (R2 = 0.982). The irrigation—fertilizer parameters (PMDI; F) and crop variety (V) are the most effective in estimating the yield and WP of tomato crops. Statistical analysis of the obtained results showed that the SO–SVR hybrid method has high efficiency in estimating WP and yield. In general, intelligent hybrid methods can enable the optimal and economical use of water and fertilizer resources. © 2021 by the authors. Licensee MDPI, Basel, Switzerland.},
note = {2},
keywords = {},
pubstate = {published},
tppubtype = {article}
}
Basu, T.; Das, A.; Pham, Q. B.; Al-Ansari, N.; Linh, N. T. T.; Lagerwall, G.
In: Scientific Reports, vol. 11, no. 1, 2021, ISSN: 20452322.
@article{2-s2.0-85102996743,
title = {Author Correction: Development of an integrated peri-urban wetland degradation assessment approach for the Chatra Wetland in eastern India (Scientific Reports, (2021), 11, 1, (4470), 10.1038/s41598-021-83512-6)},
author = { T. Basu and A. Das and Q.B. Pham and N. Al-Ansari and N.T.T. Linh and G. Lagerwall},
url = {https://www.scopus.com/inward/record.uri?eid=2-s2.0-85102996743&doi=10.1038%2fs41598-021-86634-z&partnerID=40&md5=7df21dd5a0dc20745f2c83b436c6d60d},
doi = {10.1038/s41598-021-86634-z},
issn = {20452322},
year = {2021},
date = {2021-01-01},
journal = {Scientific Reports},
volume = {11},
number = {1},
publisher = {Nature Research},
abstract = {In the original version of this Article, Nguyen Thi Thuy Linh was incorrectly affiliated with ‘Institute of Research and Development, Duy Tan University, Danang 550000, Vietnam’ and ‘Faculty of Environmental and Chemical Engineering, Duy Tan University, Danang 550000, Vietnam’. The correct affiliation is listed below. Thuyloi University, 175 Tay Son, Dong Da, Hanoi, Vietnam. Additionally, the corresponding email of Nguyen Thi Thuy Linh was incorrect. Correspondence and request for materials should be addressed to linhntt@tlu.edu.vn. These errors have now been corrected in the HTML and PDF versions of this Article, and in the accompanying Supplementary Information file. © 2021, The Author(s).},
keywords = {},
pubstate = {published},
tppubtype = {article}
}
Basu, T.; Das, A.; Pham, Q. B.; Al-Ansari, N.; Linh, N. T. T.; Lagerwall, G.
Development of an integrated peri-urban wetland degradation assessment approach for the Chatra Wetland in eastern India Journal Article
In: Scientific Reports, vol. 11, no. 1, 2021, ISSN: 20452322, (15).
@article{2-s2.0-85101517298,
title = {Development of an integrated peri-urban wetland degradation assessment approach for the Chatra Wetland in eastern India},
author = { T. Basu and A. Das and Q.B. Pham and N. Al-Ansari and N.T.T. Linh and G. Lagerwall},
url = {https://www.scopus.com/inward/record.uri?eid=2-s2.0-85101517298&doi=10.1038%2fs41598-021-83512-6&partnerID=40&md5=6c9ea4b4f913bdab325d92bdd44403b8},
doi = {10.1038/s41598-021-83512-6},
issn = {20452322},
year = {2021},
date = {2021-01-01},
journal = {Scientific Reports},
volume = {11},
number = {1},
publisher = {Nature Research},
abstract = {The loss of peri-urban wetlands is a major side effect of urbanization in India in recent days. Timely and proper assessment of wetland area change is essential for the conservation of wetlands. This study follows the integrated way of the peri-urban wetland degradation assessment in the case of medium and small-size urban agglomerations with a special focus on Chatra Wetland. Analysis of land-use and land cover (LULC) maps of the past 28 years shows a decrease of 60% area of the wetland including marshy land. This has reduced the ecosystem services value by about 71.90% over the period 1991–2018. From this end, The Land Change Modeler of IDRISI TerrSet using the combination of MLPNN and Markov Chain has been used to predict the LULC map of this region. The scenario-based modeling following the LULC conversion and nine explanatory variables suggests the complete loss of this wetland by 2045. However, the authors have also tried to present a future LULC pattern of this region based on an environmental perspective. This proposed map suggests possible areas for built-up expansion on the western side of the city without significantly affecting the environment. © 2021, The Author(s).},
note = {15},
keywords = {},
pubstate = {published},
tppubtype = {article}
}
Shahid, M.; Rahman, K. U.; Haider, S.; Gabriel, H. F.; Khan, A. J.; Pham, Q. B.; Pande, C. B.; Linh, N. T. T.; Anh, D. T.
Quantitative assessment of regional land use and climate change impact on runoff across Gilgit watershed Journal Article
In: Environmental Earth Sciences, vol. 80, no. 22, 2021, ISSN: 18666280, (11).
@article{2-s2.0-85118304932,
title = {Quantitative assessment of regional land use and climate change impact on runoff across Gilgit watershed},
author = { M. Shahid and K.U. Rahman and S. Haider and H.F. Gabriel and A.J. Khan and Q.B. Pham and C.B. Pande and N.T.T. Linh and D.T. Anh},
url = {https://www.scopus.com/inward/record.uri?eid=2-s2.0-85118304932&doi=10.1007%2fs12665-021-10032-x&partnerID=40&md5=89f1ac0485d9c0ca61ea1cd9304593e7},
doi = {10.1007/s12665-021-10032-x},
issn = {18666280},
year = {2021},
date = {2021-01-01},
journal = {Environmental Earth Sciences},
volume = {80},
number = {22},
publisher = {Springer Science and Business Media Deutschland GmbH},
abstract = {Regional climate change (CC) and land use changes (LUCs) can significantly influence the hydrological processes at watershed scale. Different studies have investigated the impact of climate change in the Indus Basin. However, there is a need to investigate the impact of environmental changes on the regional hydrology over a complex topographic region. This study quantitatively assesses the relative contributions of CC and LUC on runoff alterations across Gilgit watershed by using multivariable calibration approach using the Soil and Water Assessment Tool (SWAT). Mann–Kendall (MK) and Pettitt tests are applied to identify the trends and changes in runoff and climatic variables during 1985–2013. The supervised classification is performed to acquire land use maps and other quantitative details required for the analyses. Moreover, Indicators of Hydrologic Alterations (IHA) analyses were performed for the first time in the Gilgit watershed to investigate the impact of CC and LUCs during the pre- and post-impact periods. The results demonstrated that precipitation, temperature, and runoff of the Gilgit watershed presented significant increasing trends. The change point using Pettitt test is depicted in 1999, 1995, and 1998, respectively. The mean annual increasing rate of precipitation, temperature, and runoff is 4.92 mm/year, 0.04 °C/year, and 2.60 m3/year, respectively. SWAT model performed well and the relative attributed contribution of CC to runoff change is 97.22% and it is 2.78% for LUC. The IHA results showed that runoff has significantly increased in post-impact (1999–2013) as compared to pre-impact (1985–1998), which was further confirmed by analyzing the IHA results using percent bias (PBIAS). Significant overestimation of runoff (higher runoff in post-impact period) was observed in the wet (maximum runoff) season. This study demonstrated that the high contribution of CC to runoff change is mainly due to the change in climate variables and global warming trends. © 2021, The Author(s), under exclusive licence to Springer-Verlag GmbH Germany, part of Springer Nature.},
note = {11},
keywords = {},
pubstate = {published},
tppubtype = {article}
}
Aiyelokun, O.; Pham, Q. B.; Aiyelokun, O.; Malik, A.; Adarsh, S.; Mohammadi, B.; Linh, N. T. T.; Zakwan, M.
Credibility of design rainfall estimates for drainage infrastructures: extent of disregard in Nigeria and proposed framework for practice Journal Article
In: Natural Hazards, vol. 109, no. 2, pp. 1557-1588, 2021, ISSN: 0921030X, (1).
@article{2-s2.0-85110789908,
title = {Credibility of design rainfall estimates for drainage infrastructures: extent of disregard in Nigeria and proposed framework for practice},
author = { O. Aiyelokun and Q.B. Pham and O. Aiyelokun and A. Malik and S. Adarsh and B. Mohammadi and N.T.T. Linh and M. Zakwan},
url = {https://www.scopus.com/inward/record.uri?eid=2-s2.0-85110789908&doi=10.1007%2fs11069-021-04889-1&partnerID=40&md5=7236519ca24b2732d0531dd874a4029b},
doi = {10.1007/s11069-021-04889-1},
issn = {0921030X},
year = {2021},
date = {2021-01-01},
journal = {Natural Hazards},
volume = {109},
number = {2},
pages = {1557-1588},
publisher = {Springer Science and Business Media B.V.},
abstract = {Rainfall intensity or depth estimates are vital input for hydrologic and hydraulic models used in designing drainage infrastructures. Unfortunately, these estimates are susceptible to different sources of uncertainties including climate change, which could have high implications on the cost and design of hydraulic structures. This study adopts a systematic literature review to ascertain the disregard of credibility assessment of rainfall estimates in Nigeria. Thereafter, a simple framework for informing the practice of reliability check of rainfall estimates was proposed using freely available open-source tools and applied to the north central region of Nigeria. The study revealed through a synthesis matrix that in the last decade, both empirical and theoretical methods have been applied in predicting design rainfall intensities or depths for different frequencies across Nigeria, but none of the selected studies assessed the credibility of the design estimates. This study has established through the application of the proposed framework that drainage infrastructure designed in the study area using 100–1000-year return periods are more susceptible to error. And that the extent of the credibility of quantitative estimates of extreme rains leading to flooding is not equal for each variability indicator across a large spatial region. Hence, to optimize informed decision-making regarding flood risk reduction by risk assessor, variability and uncertainty of rainfall estimates should be assessed spatially to minimize erroneous deductions. © 2021, The Author(s), under exclusive licence to Springer Nature B.V.},
note = {1},
keywords = {},
pubstate = {published},
tppubtype = {article}
}
Linh, N. T. T.; Ruigar, H.; Golian, S.; Bawoke, G. T.; Gupta, V.; Rahman, K. U.; Adarsh, S.; Pham, Q. B.
Flood prediction based on climatic signals using wavelet neural network Journal Article
In: Acta Geophysica, vol. 69, no. 4, pp. 1413-1426, 2021, ISSN: 18956572, (8).
@article{2-s2.0-85111964528,
title = {Flood prediction based on climatic signals using wavelet neural network},
author = { N.T.T. Linh and H. Ruigar and S. Golian and G.T. Bawoke and V. Gupta and K.U. Rahman and S. Adarsh and Q.B. Pham},
url = {https://www.scopus.com/inward/record.uri?eid=2-s2.0-85111964528&doi=10.1007%2fs11600-021-00620-7&partnerID=40&md5=c3976d85cb4d62e299cc7b283bbbf1d0},
doi = {10.1007/s11600-021-00620-7},
issn = {18956572},
year = {2021},
date = {2021-01-01},
journal = {Acta Geophysica},
volume = {69},
number = {4},
pages = {1413-1426},
publisher = {Springer Science and Business Media Deutschland GmbH},
abstract = {Large-scale climatic circulation modulates the weather patterns around the world. Understanding the teleconnections between large-scale circulation and local hydro-climatological variables has been a major thrust area of hydro-climatology research. The large-scale circulation is often quantified in terms of sea surface temperature (SST) and sea-level pressure (SLP). In this paper, we investigate the potential of wavelet neural network (WNN) hybrid model to predict maximum monthly discharge of the Madarsoo watershed, North of Iran considering two large-scale climatic signals like SST and SLP as inputs. Error measures like root-mean-square error (RMSE), and mean absolute error along with the correlation measures like coefficient of correlation (R), and Nash–Sutcliffe coefficient (CNS) were used to quantify the performance of prediction of maximum monthly discharge of three different hydrometry stations of the watershed. In all the cases, the WNN hybrid machine learning model was found to be giving superior performance consistently against the standalone artificial neural network (ANN) model and multiple linear regression model to predict the flood discharges of March and August months. The prediction of flood for August which is more devastating is found to be slightly better than the prediction of floods of March, in the stations served with smaller drainage area. The RMSE, R and CNS of Tamer hydrometry station in August were found to be 0.68, 0.996, and 0.99 m3/s, respectively, for the test period by using WNN model against 1.55, 0.989 and 0.95 by ANN model. Moreover, when evaluated for predicting the maximum monthly discharge in March and August between 2012 and 2013, the wavelet-based neural networks performed remarkably well than the ANN. © 2021, Institute of Geophysics, Polish Academy of Sciences & Polish Academy of Sciences.},
note = {8},
keywords = {},
pubstate = {published},
tppubtype = {article}
}
Islam, A. R. M. T.; Talukdar, S.; Mahato, S.; Ziaul, S.; Eibek, K. U.; Akhter, S.; Pham, Q. B.; Mohammadi, B.; Karimi, F.; Linh, N. T. T.
In: Environmental Science and Pollution Research, vol. 28, no. 26, pp. 34472-, 2021, ISSN: 09441344.
@article{2-s2.0-85102800013,
title = {Correction to: Machine learning algorithm-based risk assessment of riparian wetlands in Padma River basin of Northwest Bangladesh (Environmental Science and Pollution Research, (2021), 28, 26, (34450-34471), 10.1007/s11356-021-12806-z)},
author = { A.R.M.T. Islam and S. Talukdar and S. Mahato and S. Ziaul and K.U. Eibek and S. Akhter and Q.B. Pham and B. Mohammadi and F. Karimi and N.T.T. Linh},
url = {https://www.scopus.com/inward/record.uri?eid=2-s2.0-85102800013&doi=10.1007%2fs11356-021-13480-x&partnerID=40&md5=f26f7403cd7a575d41f6fd5806a1523b},
doi = {10.1007/s11356-021-13480-x},
issn = {09441344},
year = {2021},
date = {2021-01-01},
journal = {Environmental Science and Pollution Research},
volume = {28},
number = {26},
pages = {34472-},
publisher = {Springer Science and Business Media Deutschland GmbH},
abstract = {The correct affiliation of the last Author is shown in this paper. The original article has been corrected. © 2021, Springer-Verlag GmbH Germany, part of Springer Nature.},
keywords = {},
pubstate = {published},
tppubtype = {article}
}
Islam, A. R. M. T.; Talukdar, S.; Mahato, S.; Ziaul, S.; Eibek, K. U.; Akhter, S.; Pham, Q. B.; Mohammadi, B.; Karimi, F.; Linh, N. T. T.
Machine learning algorithm-based risk assessment of riparian wetlands in Padma River Basin of Northwest Bangladesh Journal Article
In: Environmental Science and Pollution Research, vol. 28, no. 26, pp. 34450-34471, 2021, ISSN: 09441344, (29).
@article{2-s2.0-85102081024,
title = {Machine learning algorithm-based risk assessment of riparian wetlands in Padma River Basin of Northwest Bangladesh},
author = { A.R.M.T. Islam and S. Talukdar and S. Mahato and S. Ziaul and K.U. Eibek and S. Akhter and Q.B. Pham and B. Mohammadi and F. Karimi and N.T.T. Linh},
url = {https://www.scopus.com/inward/record.uri?eid=2-s2.0-85102081024&doi=10.1007%2fs11356-021-12806-z&partnerID=40&md5=b609374b7bfbf04104a15f2e7956d3b7},
doi = {10.1007/s11356-021-12806-z},
issn = {09441344},
year = {2021},
date = {2021-01-01},
journal = {Environmental Science and Pollution Research},
volume = {28},
number = {26},
pages = {34450-34471},
publisher = {Springer Science and Business Media Deutschland GmbH},
abstract = {Wetland risk assessment is a global concern especially in developing countries like Bangladesh. The present study explored the spatiotemporal dynamics of wetlands, prediction of wetland risk assessment. The wetland risk assessment was predicted based on ten selected parameters, such as fragmentation probability, distance to road, and settlement. We used M5P, random forest (RF), reduced error pruning tree (REPTree), and support vector machine (SVM) machine learning techniques for wetland risk assessment. The results showed that wetland areas at present are declining less than one-third of those in 1988 due to the construction of the dam at Farakka, which is situated at the upstream of the Padma River. The distance to the river and built-up area are the two most contributing drivers influencing the wetland risk assessment based on information gain ratio (InGR). The prediction results of machine learning models showed 64.48% of area by M5P, 61.75% of area by RF, 62.18% of area by REPTree, and 55.74% of area by SVM have been predicted as the high and very high-risk zones. The results of accuracy assessment showed that the RF outperformed than other models (area under curve: 0.83), followed by the SVM, M5P, and REPTree. Degradation of wetlands explored in this study demonstrated the negative effects on biodiversity. Therefore, to conserve and protect the wetlands, continuous monitoring of wetlands using high resolution satellite images, feeding with the ecological flow, confining built up area and agricultural expansion towards wetlands, and new wetland creation is essential for wetland management. Graphical abstract: [Figure not available: see fulltext.] © 2021, The Author(s), under exclusive licence to Springer-Verlag GmbH, DE part of Springer Nature.},
note = {29},
keywords = {},
pubstate = {published},
tppubtype = {article}
}
Tariq, A.; Shu, H.; Kuriqi, A.; Siddiqui, S.; Gagnon, A. S.; Lu, L.; Linh, N. T. T.; Pham, Q. B.
Characterization of the 2014 indus river flood using hydraulic simulations and satellite images Journal Article
In: Remote Sensing, vol. 13, no. 11, 2021, ISSN: 20724292, (20).
@article{2-s2.0-85107304048,
title = {Characterization of the 2014 indus river flood using hydraulic simulations and satellite images},
author = { A. Tariq and H. Shu and A. Kuriqi and S. Siddiqui and A.S. Gagnon and L. Lu and N.T.T. Linh and Q.B. Pham},
url = {https://www.scopus.com/inward/record.uri?eid=2-s2.0-85107304048&doi=10.3390%2frs13112053&partnerID=40&md5=933b6f5d3c1f9a8e5a869efd1998eec8},
doi = {10.3390/rs13112053},
issn = {20724292},
year = {2021},
date = {2021-01-01},
journal = {Remote Sensing},
volume = {13},
number = {11},
publisher = {MDPI AG},
abstract = {Rivers play an essential role to humans and ecosystems, but they also burst their banks during floods, often causing extensive damage to crop, property, and loss of lives. This paper characterizes the 2014 flood of the Indus River in Pakistan using the US Army Corps of Engineers Hy-drologic Engineering Centre River Analysis System (HEC-RAS) model, integrated into a geographic information system (GIS) and satellite images from Landsat-8. The model is used to estimate the spatial extent of the flood and assess the damage that it caused by examining changes to the different land-use/land-cover (LULC) types of the river basin. Extreme flows for different return periods were estimated using a flood frequency analysis using a log-Pearson III distribution, which the Kolmogorov–Smirnov (KS) test identified as the best distribution to characterize the flow regime of the Indus River at Taunsa Barrage. The output of the flood frequency analysis was then incorporated into the HEC-RAS model to determine the spatial extent of the 2014 flood, with the accuracy of this modelling approach assessed using images from the Moderate Resolution Imaging Spectroradiometer (MODIS). The results show that a supervised classification of the Landsat images was able to identify the LULC types of the study region with a high degree of accuracy, and that the most affected LULC was crop/agricultural land, of which 50% was affected by the 2014 flood. Finally, the hydraulic simulation of extent of the 2014 flood was found to visually compare very well with the MODIS image, and the surface area of floods of different return periods was calculated. This paper provides further evidence of the benefit of using a hydrological model and satellite images for flood mapping and for flood damage assessment to inform the development of risk mitigation strategies. © 2021 by the authors. Licensee MDPI, Basel, Switzerland.},
note = {20},
keywords = {},
pubstate = {published},
tppubtype = {article}
}
Elkhrachy, I.; Pham, Q. B.; Costache, R.; Mohajane, M.; Rahman, K. U.; Shahabi, H.; Linh, N. T. T.; Anh, D. T.
In: Journal of Flood Risk Management, vol. 14, no. 2, 2021, ISSN: 1753318X, (10).
@article{2-s2.0-85100857382,
title = {Sentinel-1 remote sensing data and Hydrologic Engineering Centres River Analysis System two-dimensional integration for flash flood detection and modelling in New Cairo City, Egypt},
author = { I. Elkhrachy and Q.B. Pham and R. Costache and M. Mohajane and K.U. Rahman and H. Shahabi and N.T.T. Linh and D.T. Anh},
url = {https://www.scopus.com/inward/record.uri?eid=2-s2.0-85100857382&doi=10.1111%2fjfr3.12692&partnerID=40&md5=6eedfb5b46cdf08add2bcfd5868ead25},
doi = {10.1111/jfr3.12692},
issn = {1753318X},
year = {2021},
date = {2021-01-01},
journal = {Journal of Flood Risk Management},
volume = {14},
number = {2},
publisher = {Blackwell Publishing Inc.},
abstract = {Digital surface models, land use and rainfall data were used to simulate water areas using Hydrologic Engineering Centres River Analysis System (HEC-RAS) software. Multi-temporal synthetic aperture radar (SAR) was used for the detection of flood prone area to calibrate HEC-RAS, due to the lack of data validation in the New Cairo City, Egypt. The thresholding water detection method was applied to two Sentinel-1 images, one pre- and one post-flash flood event from April 24 to 27, 2018. The threshold method was used to detect water areas from SAR Sentinel-1 images. Feature statistical agreement F1 and F2 values ranged from 73.4 to 77.7% between water areas extracted based on backscattering values between 19.97 and 16.53 in decibels (dB) and reference water areas obtained using an optical image of the Sentinel-2 satellite. The similarity between simulated HEC-RAS two-dimensional (2D) of water areas and reference water areas based on SAR data ranged between 74.2 and 89.7% using feature statistical agreement values F1 and F2. It provides a clear suggestion that, in the absence of field observations, SAR data can be used to calibrate the model. Two flood hazard maps created based on water velocity and depth were obtained from HEC-RAS 2D simulation. The obtained maps indicated that 11% of the roads and 50% of the buildings in New Cairo City are exposed to high hazard areas. Furthermore, 28% of the bare land is situated in a very high vulnerability area. We recommend the use of obtained hazard map in supporting emergency response, and designing effective emergency plans. © 2021 The Authors. Journal of Flood Risk Management published by Chartered Institution of Water and Environmental Management and John Wiley & Sons Ltd.},
note = {10},
keywords = {},
pubstate = {published},
tppubtype = {article}
}
Mohammadi, B.; Mehdizadeh, S.; Ahmadi, F.; Lien, N. T. T.; Linh, N. T. T.; Pham, Q. B.
Developing hybrid time series and artificial intelligence models for estimating air temperatures Journal Article
In: Stochastic Environmental Research and Risk Assessment, vol. 35, no. 6, pp. 1189-1204, 2021, ISSN: 14363240, (11).
@article{2-s2.0-85092801511,
title = {Developing hybrid time series and artificial intelligence models for estimating air temperatures},
author = { B. Mohammadi and S. Mehdizadeh and F. Ahmadi and N.T.T. Lien and N.T.T. Linh and Q.B. Pham},
url = {https://www.scopus.com/inward/record.uri?eid=2-s2.0-85092801511&doi=10.1007%2fs00477-020-01898-7&partnerID=40&md5=473ae66e5b72a849d24aa8ebf58d2a97},
doi = {10.1007/s00477-020-01898-7},
issn = {14363240},
year = {2021},
date = {2021-01-01},
journal = {Stochastic Environmental Research and Risk Assessment},
volume = {35},
number = {6},
pages = {1189-1204},
publisher = {Springer Science and Business Media Deutschland GmbH},
abstract = {Air temperature is a vital meteorological variable required in many applications, such as agricultural and soil sciences, meteorological and climatological studies, etc. Given the importance of this variable, this study seeks to estimate minimum (Tmin), maximum (Tmax), and mean (T) air temperatures by applying a linear autoregressive (AR) time series model and then developing a hybrid model by means of coupling the AR and a non-linear time series model, namely autoregressive conditional heteroscedasticity (ARCH). Hence, the hybrid AR-ARCH model was tested. To that end, the Tmin, Tmax, and T data from 1986 to 2015 at two weather stations located in Northwestern Iran were used for both daily and monthly time scales. The results showed that the hybrid time series model (i.e.; AR-ARCH) performed better than the single AR for estimating the air temperature parameters at the study sites. Multi-layer perceptron (MLP) was then employed to estimate the air temperatures using lagged temperature data as input predictors. Next, the single AR and hybrid AR-ARCH time series models were utilized to implement the hybrid MLP-AR and MLP-AR-ARCH models. It is worth noting that developing the hybrid MLP-AR and MLP-AR-ARCH models, as well as AR-ARCH one is the novelty of this study. Three statistical metrics including root mean square error (RMSE), mean absolute error (MAE), and normalized RMSE (NRMSE) were used to investigate the performance of whole the developed models. The hybrid MLP-AR and MLP-AR-ARCH models were found to perform better than the single MLP when estimating the daily and monthly Tmin, Tmax, and T; however, the MLP-AR models outperformed the MLP-AR-ARCH ones. At the end of this study, the performance of MLP was evaluated under an external condition (i.e.; estimating the temperature components at any particular site using the temperature data of an adjacent location). The results indicated that the temperature data of a nearby station can be used for estimating the temperatures of a desired station. Most accurate results during the test stage were obtained under a local assessment through the hybrid MLP-AR(1) at the Tabriz station when estimating the monthly Tmax (RMSE = 0.199 °C; MAE = 0.159 °C; NRMSE = 1.012%) and hybrid MLP-AR(12) at the Urmia station when estimating the daily Tmax (RMSE = 0.364 °C; MAE = 0.277 °C; NRMSE = 1.911%). © 2020, Springer-Verlag GmbH Germany, part of Springer Nature.},
note = {11},
keywords = {},
pubstate = {published},
tppubtype = {article}
}
Şan, M.; Akçay, F.; Linh, N. T. T.; Kankal, M.; Pham, Q. B.
Innovative and polygonal trend analyses applications for rainfall data in Vietnam Journal Article
In: Theoretical and Applied Climatology, vol. 144, no. 3-4, pp. 809-822, 2021, ISSN: 0177798X, (12).
@article{2-s2.0-85101941818,
title = {Innovative and polygonal trend analyses applications for rainfall data in Vietnam},
author = { M. Şan and F. Akçay and N.T.T. Linh and M. Kankal and Q.B. Pham},
url = {https://www.scopus.com/inward/record.uri?eid=2-s2.0-85101941818&doi=10.1007%2fs00704-021-03574-4&partnerID=40&md5=f421d7bd55c529f5f8d50f55bbcbcf99},
doi = {10.1007/s00704-021-03574-4},
issn = {0177798X},
year = {2021},
date = {2021-01-01},
journal = {Theoretical and Applied Climatology},
volume = {144},
number = {3-4},
pages = {809-822},
publisher = {Springer},
abstract = {It is a known fact that the size, frequency, and spatial variability of hydrometeorological variables will irregularly increase under the impact of climate change. Among the hydrometeorological variables, rainfall is one of the most important. Trend analysis is one of the most effective methods of observing the effects of climate change on rainfall. Recently, new graphical methods have been proposed as an alternative to classical trend analysis methods. Innovative Polygon Trend Analysis (IPTA), which evolved from Innovative Trend Analysis (ITA), is currently one of the proposed methods and it does not contain any assumptions. The aim of this study is to compare IPTA, ITA with the Significance Test and Mann-Kendall (MK) methods. To achieve this, the monthly total rainfall trends of 15 stations in the Vu Gia-Thu Bon River Basin (VGTBRB) of Vietnam have been examined for the period 1979–2016. The analyses show that rainfall tends to increase (decrease) in March (June) at nearly all stations. IPTA and ITA with the Significance Test are more sensitive than MK in determining the trends. While trends were detected in approximately 90% of all months in IPTA and ITA with the Significance Test, this rate was only 23% in the MK test. Although the arithmetic mean graphs in the 1-year hydrometeorological cycle are considerably regular at almost all stations, their standard deviations are relatively irregular. The most critical month for trend transitions between consecutive months for all the stations is October, which has an average trend slope of −1.35 and a trend slope ranging from −3.98 to −0.21, which shows a decreasing trend. © 2021, The Author(s), under exclusive licence to Springer-Verlag GmbH, AT part of Springer Nature.},
note = {12},
keywords = {},
pubstate = {published},
tppubtype = {article}
}
Islam, A. R. M. T.; Talukdar, S.; Mahato, S.; Kundu, S.; Eibek, K. U.; Pham, Q. B.; Kuriqi, A.; Linh, N. T. T.
Flood susceptibility modelling using advanced ensemble machine learning models Journal Article
In: Geoscience Frontiers, vol. 12, no. 3, 2021, ISSN: 16749871, (119).
@article{2-s2.0-85099699170,
title = {Flood susceptibility modelling using advanced ensemble machine learning models},
author = { A.R.M.T. Islam and S. Talukdar and S. Mahato and S. Kundu and K.U. Eibek and Q.B. Pham and A. Kuriqi and N.T.T. Linh},
url = {https://www.scopus.com/inward/record.uri?eid=2-s2.0-85099699170&doi=10.1016%2fj.gsf.2020.09.006&partnerID=40&md5=0c12f4e90040d8f4d3d85e7da7db2665},
doi = {10.1016/j.gsf.2020.09.006},
issn = {16749871},
year = {2021},
date = {2021-01-01},
journal = {Geoscience Frontiers},
volume = {12},
number = {3},
publisher = {Elsevier B.V.},
abstract = {Because of the tremendous damage to properties, infrastructures, and human casualties, floods are one of the greatest devastating disasters from nature. Due to the dynamic and complex nature of the flash flood, it is challenging to predict the sites which are vulnerable to flash floods. Therefore, earlier identification of flash flood susceptible sites can be performed using advanced machine learning models for managing flood disasters. In this study, we applied and assessed two new hybrid ensemble models, namely Dagging and Random Subspace (RS) coupled with Artificial Neural Network (ANN), Random Forest (RF), and Support Vector Machine (SVM) which are the other three state-of-the-art machine learning models for modelling flood susceptibility maps at the Teesta River basin, the northern region of Bangladesh. The application of these models includes twelve flood influencing factors with 413 current and former flooding points, which were transferred in a GIS environment. The information gain ratio, the multicollinearity diagnostics tests were employed to determine the association between the occurrences and flood influential factors. For the validation and the comparison of these models, for the ability to predict the statistical appraisal measures such as Freidman, Wilcoxon signed-rank, and t-paired tests and Receiver Operating Characteristic Curve (ROC) were employed. The value of the Area Under the Curve (AUC) of ROC was above 0.80 for all models. For flood susceptibility modelling, the Dagging model performs superior, followed by RF, the ANN, the SVM, and the RS, then the several benchmark models. The methodology and solution-oriented results presented in this paper will assist the regional as well as local authorities and the policy-makers for mitigating the risks related to floods and also help in developing appropriate mitigation measures to avoid potential damages. © 2020 Elsevier B.V.},
note = {119},
keywords = {},
pubstate = {published},
tppubtype = {article}
}
Singh, V. K.; Kumar, D.; Singh, S. K.; Pham, Q. B.; Linh, N. T. T.; Mohammed, S.; Anh, D. T.
Development of fuzzy analytic hierarchy process based water quality model of Upper Ganga river basin, India Journal Article
In: Journal of Environmental Management, vol. 284, 2021, ISSN: 03014797, (9).
@article{2-s2.0-85100703494,
title = {Development of fuzzy analytic hierarchy process based water quality model of Upper Ganga river basin, India},
author = { V.K. Singh and D. Kumar and S.K. Singh and Q.B. Pham and N.T.T. Linh and S. Mohammed and D.T. Anh},
url = {https://www.scopus.com/inward/record.uri?eid=2-s2.0-85100703494&doi=10.1016%2fj.jenvman.2021.111985&partnerID=40&md5=09ec916e9825e704137ffe6a05f84a4f},
doi = {10.1016/j.jenvman.2021.111985},
issn = {03014797},
year = {2021},
date = {2021-01-01},
journal = {Journal of Environmental Management},
volume = {284},
publisher = {Academic Press},
abstract = {The ecological sustainability of rivers is in question due to severe pollution and lack of stringent regulations. Long term (1990–2016) water quality data of five stations namely Haridwar, Bareilly, Kanpur, Prayagraj and Varanasi of Upper Ganga river, India was considered for analysis using fuzzy analytical process (FAHP) based water quality index (WQI) to assess surface water quality. The value of water physical, biological and chemical parameters of temporal resolution (monthly; seasonal and yearly) indicate that value of electrical conductivity (EC), total dissolved solids (TDS), biological oxygen demand (BOD), chemical oxygen demand (COD), total alkalinity (Mg CaCO3), total hardness (Mg CaCO3), calcium (Ca), magnesium (Mg), sodium (Na), chlorine (Cl) and bicarbonate (HCO3) were observed very high compared to recommended value of Bureau of Indian Standards (BIS) and World Health Organization (WHO) at Kanpur, Prayagraj and Varanasi stations. However, low value of parameters is observed at Haridwar and Bareilly stations. Also, the high deviation was observed in water quality parameters during 1990–2010 whereas the deviation of parameters is decreased in 2011–2016. It is observed from the piper diagram that magnesium and bicarbonate at Haridwar, sodium, potassium and bicarbonate in Bareilly, Kanpur, Prayagraj and Varanasi stations are dominant during monthly and seasonal periods. The fuzzy based WQI value indicate that water quality is excellent to poor at Haridwar, while poor to unsuitable in Bareilly, Kanpur, Prayagraj and Varanasi during monthly and seasonal periods. The water quality ranges from poor to unsuitable during the 1990–2010 period and good to very poor during the 2011–2016 period at Bareilly, Kanpur, Prayagraj and Varanasi stations. Whereas very good to good during 1990–2010 and excellent to good during 2011–2016 at Haridwar. It was also determined that water quality parameters (Ca; Na+K; SO4; Hardness; Cl and Mg) and WQI values were increased with length of the stream. It indicates that drain discharge, urban growth, urban functions, ecological footprints and crop area increment were key sources of pollution. © 2021 Elsevier Ltd},
note = {9},
keywords = {},
pubstate = {published},
tppubtype = {article}
}
Ali, S. A.; Parvin, F.; Vojteková, J.; Costache, R.; Linh, N. T. T.; Pham, Q. B.; Vojtek, M.; Gigović, L.; Ahmad, A.; Ghorbani, M. A.
GIS-based landslide susceptibility modeling: A comparison between fuzzy multi-criteria and machine learning algorithms Journal Article
In: Geoscience Frontiers, vol. 12, no. 2, pp. 857-876, 2021, ISSN: 16749871, (45).
@article{2-s2.0-85098724608,
title = {GIS-based landslide susceptibility modeling: A comparison between fuzzy multi-criteria and machine learning algorithms},
author = { S.A. Ali and F. Parvin and J. Vojteková and R. Costache and N.T.T. Linh and Q.B. Pham and M. Vojtek and L. Gigović and A. Ahmad and M.A. Ghorbani},
url = {https://www.scopus.com/inward/record.uri?eid=2-s2.0-85098724608&doi=10.1016%2fj.gsf.2020.09.004&partnerID=40&md5=458f533ef1f353f6a81e4756e0a16707},
doi = {10.1016/j.gsf.2020.09.004},
issn = {16749871},
year = {2021},
date = {2021-01-01},
journal = {Geoscience Frontiers},
volume = {12},
number = {2},
pages = {857-876},
publisher = {Elsevier B.V.},
abstract = {Hazards and disasters have always negative impacts on the way of life. Landslide is an overwhelming natural as well as man-made disaster that causes loss of natural resources and human properties throughout the world. The present study aimed to assess and compare the prediction efficiency of different models in landslide susceptibility in the Kysuca river basin, Slovakia. In this regard, the fuzzy decision-making trial and evaluation laboratory combining with the analytic network process (FDEMATEL-ANP), Naïve Bayes (NB) classifier, and random forest (RF) classifier were considered. Initially, a landslide inventory map was produced with 2000 landslide and non-landslide points by randomly divided with a ratio of 70%:30% for training and testing, respectively. The geospatial database for assessing the landslide susceptibility was generated with the help of 16 landslide conditioning factors by allowing for topographical, hydrological, lithological, and land cover factors. The ReliefF method was considered for determining the significance of selected conditioning factors and inclusion in the model building. Consequently, the landslide susceptibility maps (LSMs) were generated using the FDEMATEL-ANP, Naïve Bayes (NB) classifier, and random forest (RF) classifier models. Finally, the area under curve (AUC) and different arithmetic evaluation were used for validating and comparing the results and models. The results revealed that random forest (RF) classifier is a promising and optimum model for landslide susceptibility in the study area with a very high value of area under curve (AUC = 0.954), lower value of mean absolute error (MAE = 0.1238) and root mean square error (RMSE = 0.2555), and higher value of Kappa index (K = 0.8435) and overall accuracy (OAC = 92.2%). © 2020 Elsevier B.V.},
note = {45},
keywords = {},
pubstate = {published},
tppubtype = {article}
}
Ahmadlou, M.; Al-Fugara, A.; Al-Shabeeb, A. R.; Arora, A.; Al-Adamat, R.; Pham, Q. B.; Al-Ansari, N.; Linh, N. T. T.; Sajedi, H.
Flood susceptibility mapping and assessment using a novel deep learning model combining multilayer perceptron and autoencoder neural networks Journal Article
In: Journal of Flood Risk Management, vol. 14, no. 1, 2021, ISSN: 1753318X, (32).
@article{2-s2.0-85097774261,
title = {Flood susceptibility mapping and assessment using a novel deep learning model combining multilayer perceptron and autoencoder neural networks},
author = { M. Ahmadlou and A. Al-Fugara and A.R. Al-Shabeeb and A. Arora and R. Al-Adamat and Q.B. Pham and N. Al-Ansari and N.T.T. Linh and H. Sajedi},
url = {https://www.scopus.com/inward/record.uri?eid=2-s2.0-85097774261&doi=10.1111%2fjfr3.12683&partnerID=40&md5=ef39602d47ce26dda29d775c2f10b71b},
doi = {10.1111/jfr3.12683},
issn = {1753318X},
year = {2021},
date = {2021-01-01},
journal = {Journal of Flood Risk Management},
volume = {14},
number = {1},
publisher = {Blackwell Publishing Inc.},
abstract = {Floods are one of the most destructive natural disasters causing financial damages and casualties every year worldwide. Recently, the combination of data-driven techniques with remote sensing (RS) and geographical information systems (GIS) has been widely used by researchers for flood susceptibility mapping. This study presents a novel hybrid model combining the multilayer perceptron (MLP) and autoencoder models to produce the susceptibility maps for two study areas located in Iran and India. For two cases, nine, and twelve factors were considered as the predictor variables for flood susceptibility mapping, respectively. The prediction capability of the proposed hybrid model was compared with that of the traditional MLP model through the area under the receiver operating characteristic (AUROC) criterion. The AUROC curve for the MLP and autoencoder-MLP models were, respectively, 75 and 90, 74 and 93% in the training phase and 60 and 91, 81 and 97% in the testing phase, for Iran and India cases, respectively. The results suggested that the hybrid autoencoder-MLP model outperformed the MLP model and, therefore, can be used as a powerful model in other studies for flood susceptibility mapping. © 2020 The Authors. Journal of Flood Risk Management published by Chartered Institution of Water and Environmental Management and John Wiley & Sons Ltd.},
note = {32},
keywords = {},
pubstate = {published},
tppubtype = {article}
}
Sahoo, S.; Chakraborty, S.; Pham, Q. B.; Sharifi, E.; Sammen, S. S.; Vojtek, M.; Vojteková, J.; Elkhrachy, I.; Costache, R.; Linh, N. T. T.
In: Acta Geophysica, vol. 69, no. 1, pp. 175-198, 2021, ISSN: 18956572, (7).
@article{2-s2.0-85098735146,
title = {Recognition of district-wise groundwater stress zones using the GLDAS-2 catchment land surface model during lean season in the Indian state of West Bengal},
author = { S. Sahoo and S. Chakraborty and Q.B. Pham and E. Sharifi and S.S. Sammen and M. Vojtek and J. Vojteková and I. Elkhrachy and R. Costache and N.T.T. Linh},
url = {https://www.scopus.com/inward/record.uri?eid=2-s2.0-85098735146&doi=10.1007%2fs11600-020-00509-x&partnerID=40&md5=f99890b9f841aac8cfb1628b4288be15},
doi = {10.1007/s11600-020-00509-x},
issn = {18956572},
year = {2021},
date = {2021-01-01},
journal = {Acta Geophysica},
volume = {69},
number = {1},
pages = {175-198},
publisher = {Springer Science and Business Media Deutschland GmbH},
abstract = {Water is essential for irrigation, drinking and industrial purposes from global to the regional scale. The groundwater considered a significant water resource specifically in regions where the surface water is not sufficient. Therefore, the research problem is focused on district-wise sustainable groundwater management due to urbanization. The number of impervious surface areas like roofing on built-up areas, concrete and asphalt road surface were increased due to the level of urban development. Thus, these surface areas can inhibit infiltration and surface retention by the impact of urbanization because vegetation/forest areas are decreased. The present research examines the district-wise spatiotemporal groundwater storage (GWS) changes under terrestrial water storage using the global land data assimilation system-2 (GLDAS-2) catchment land surface model (CLSM) from 2000 to 2014 in West Bengal, India. The objective of the research is mainly focused on the delineation of groundwater stress zones (GWSZs) based on ten biophysical and hydrological factors according to the deficiency of groundwater storage using the analytic hierarchy process by the GIS platform. Additionally, the spatiotemporal soil moisture (surface soil moisture; root zone soil moisture; and profile soil moisture) changes for the identification of water stress areas using CLSM were studied. Finally, generated results were validated by the observed groundwater level and groundwater recharge data. The sensitivity analysis has been performed for GWSZs mapping due to the deficit of groundwater storage. Three correlation coefficient methods (Kendall; Pearson and Spearman) are applied for the interrelationship between the most significant parameters for the generation of GWSZ from sensitivity analysis. The results show that the northeastern (max: 1097.35 mm) and the southern (max: 993.22 mm) parts have high groundwater storage due to higher amount of soil moisture and forest cover compared to other parts of the state. The results also show that the maximum and minimum total annual groundwater recharge shown in Paschim Medinipore [(361;148.51 hectare-meter (ham)] and Howrah (31;510.46 ham) from 2012 to 2013. The generated outcome can create the best sustainable groundwater management practices based upon the human attitude toward risk. © 2021; Institute of Geophysics; Polish Academy of Sciences & Polish Academy of Sciences.},
note = {7},
keywords = {},
pubstate = {published},
tppubtype = {article}
}
Shahid, M.; Rahman, K. U.; Haider, S.; Gabriel, H. F.; Khan, A. J.; Pham, Q. B.; Mohammadi, B.; Linh, N. T. T.; Anh, D. T.
Assessing the potential and hydrological usefulness of the CHIRPS precipitation dataset over a complex topography in Pakistan Journal Article
In: Hydrological Sciences Journal, vol. 66, no. 11, pp. 1664-1684, 2021, ISSN: 02626667, (5).
@article{2-s2.0-85114445879,
title = {Assessing the potential and hydrological usefulness of the CHIRPS precipitation dataset over a complex topography in Pakistan},
author = { M. Shahid and K.U. Rahman and S. Haider and H.F. Gabriel and A.J. Khan and Q.B. Pham and B. Mohammadi and N.T.T. Linh and D.T. Anh},
url = {https://www.scopus.com/inward/record.uri?eid=2-s2.0-85114445879&doi=10.1080%2f02626667.2021.1957476&partnerID=40&md5=93ec23dc788f20fa68f024beca1f16e7},
doi = {10.1080/02626667.2021.1957476},
issn = {02626667},
year = {2021},
date = {2021-01-01},
journal = {Hydrological Sciences Journal},
volume = {66},
number = {11},
pages = {1664-1684},
publisher = {Taylor and Francis Ltd.},
abstract = {This study evaluates the spatial and temporal performance of the Climate Hazard Group InfraRed Precipitation Satellite (CHIRPS) against Tropical Rainfall Measuring Mission (TRMM) Multi-Satellite Precipitation Analysis (TMPA) 3B42/3B43 v. 7 and Global Precipitation Measurement (GPM)-based Integrated Multi-Satellite Retrievals for GPM (IMERG V06), from 2000 to 2013. Several statistical metrics were used to assess the performance of CHIRPS over the Indus Basin, and its hydrological utility is also assessed using the Soil and Water Assessment Tool (SWAT). The Gilgit and Soan basins were selected for hydrological modelling. The results demonstrate the spatial and temporal dependency of CHIRPS, i.e. better performance was observed in the Lower Indus Basin (LIB) while poor performance was observed in the Upper Indus Basin (UIB). The hydrological assessment of CHIRPS revealed poor performance (overestimation of streamflow) across the Gilgit Basin during both calibration and validation periods. Satisfactory to good performance was obtained across the Soan Basin. © 2021 IAHS.},
note = {5},
keywords = {},
pubstate = {published},
tppubtype = {article}
}
Ilyas, A. M.; Pham, Q. B.; Zhu, D.; Elahi, E.; Linh, N. T. T.; Anh, D. T.; Khedher, K. M.; Ahmadlou, M.
Multi sources hydrological assessment over Vu Gia Thu Bon Basin, Vietnam Journal Article
In: Hydrological Sciences Journal, vol. 66, no. 8, pp. 1383-1392, 2021, ISSN: 02626667, (1).
@article{2-s2.0-85109960281,
title = {Multi sources hydrological assessment over Vu Gia Thu Bon Basin, Vietnam},
author = { A.M. Ilyas and Q.B. Pham and D. Zhu and E. Elahi and N.T.T. Linh and D.T. Anh and K.M. Khedher and M. Ahmadlou},
url = {https://www.scopus.com/inward/record.uri?eid=2-s2.0-85109960281&doi=10.1080%2f02626667.2021.1935964&partnerID=40&md5=20f3b3db511e691650db32eb0ceb3288},
doi = {10.1080/02626667.2021.1935964},
issn = {02626667},
year = {2021},
date = {2021-01-01},
journal = {Hydrological Sciences Journal},
volume = {66},
number = {8},
pages = {1383-1392},
publisher = {Taylor and Francis Ltd.},
abstract = {The study aims to evaluate the long-term accuracy of global precipitation (Climate Prediction Center (CPC) and Precipitation Estimation from Remotely Sensed Information using Artificial Neural Networks–Climate Data Record (PERSIANN-CDR)) along with raingauge datasets at multiple temporal scales in the Vu Gia Thu Bon basin, Vietnam. Since there are few rainfall stations in this basin, it is important to validate multisource data for multiple purposes. This is the first time that a lumped hydrological model (i.e. Probability Distributed Moisture (PDM)) has been used for this basin. Various statistical indicators, including the correlation coefficient, mean absolute error (MAE), root mean square error (RMSE), percent bias (BIAS) and Taylor diagram, were used to evaluate the applicability of the global precipitation data and the PDM model. The precipitation datasets showed a good correlation with the raingauge rainfall data. In contrast, CPC underestimates while PERSIANN-CDR overestimates the raingauge rainfall. In general, PERSIANN-CDR performed slightly better than CPC. The daily streamflow simulation driven by PDM and all data sources underestimates the actual flow. © 2021 IAHS.},
note = {1},
keywords = {},
pubstate = {published},
tppubtype = {article}
}
Vojtek, M.; Vojteková, J.; Costache, R.; Pham, Q. B.; Lee, S.; Arshad, A.; Sahoo, S.; Linh, N. T. T.; Anh, D. T.
In: Geomatics, Natural Hazards and Risk, vol. 12, no. 1, pp. 1153-1180, 2021, ISSN: 19475705, (13).
@article{2-s2.0-85105877250,
title = {Comparison of multi-criteria-analytical hierarchy process and machine learning-boosted tree models for regional flood susceptibility mapping: a case study from Slovakia},
author = { M. Vojtek and J. Vojteková and R. Costache and Q.B. Pham and S. Lee and A. Arshad and S. Sahoo and N.T.T. Linh and D.T. Anh},
url = {https://www.scopus.com/inward/record.uri?eid=2-s2.0-85105877250&doi=10.1080%2f19475705.2021.1912835&partnerID=40&md5=3f6bf16880770cbd228f0ad49f4e7808},
doi = {10.1080/19475705.2021.1912835},
issn = {19475705},
year = {2021},
date = {2021-01-01},
journal = {Geomatics, Natural Hazards and Risk},
volume = {12},
number = {1},
pages = {1153-1180},
publisher = {Taylor and Francis Ltd.},
abstract = {Identification of areas susceptible to floods is an important issue which requires an increased attention due to the changing frequency and magnitude of floods, which is mainly a result of the ongoing climate change and increasing anthropic pressure on the landscape. In this study, the aim was to identify the areas susceptible to floods using and comparing two different approaches, namely the multi-criteria decision analysis-analytical hierarchy process (MCDA-AHP) and the machine learning-boosted classification (BCT) and boosted regression (BRT) tree. The study area was represented by the Topľa river basin, Slovakia. Altogether, seven relevant flood conditioning factors: elevation, slope, river network density, distance from river, flow accumulation, curve numbers and lithology as well as flood inventory database consisting of 107 flood locations were used. Based on the results, almost 40% of the study area is characterized by high to very high flood susceptibility using the MCDA-AHP. In case of the BCT and BRT models, the share of high and very high flood susceptibility class on the basin area is 45% and 38%, respectively. Validation of the performed flood susceptibility models confirmed generally higher accuracy of the machine learning models. The accuracy of the MCDA-AHP model was 81.33% while the accuracy of the boosted tree models was 87.70% and 91.42%, respectively, for classification and regression. The results of this study can enhance more effective preliminary flood risk assessment according to the EU Floods Directive. © 2021 The Author(s). Published by Informa UK Limited, trading as Taylor & Francis Group.},
note = {13},
keywords = {},
pubstate = {published},
tppubtype = {article}
}
Costache, R.; Arabameri, A.; Blaschke, T.; Pham, Q. B.; Pham, B. T.; Pandey, M.; Arora, A.; Linh, N. T. T.; Costache, I.
Flash-flood potential mapping using deep learning, alternating decision trees and data provided by remote sensing sensors Journal Article
In: Sensors (Switzerland), vol. 21, no. 1, pp. 1-21, 2021, ISSN: 14248220, (21).
@article{2-s2.0-85099456025,
title = {Flash-flood potential mapping using deep learning, alternating decision trees and data provided by remote sensing sensors},
author = { R. Costache and A. Arabameri and T. Blaschke and Q.B. Pham and B.T. Pham and M. Pandey and A. Arora and N.T.T. Linh and I. Costache},
url = {https://www.scopus.com/inward/record.uri?eid=2-s2.0-85099456025&doi=10.3390%2fs21010280&partnerID=40&md5=1fb6ef1ecb60687bd61d808e5aa71fd7},
doi = {10.3390/s21010280},
issn = {14248220},
year = {2021},
date = {2021-01-01},
journal = {Sensors (Switzerland)},
volume = {21},
number = {1},
pages = {1-21},
publisher = {MDPI AG},
abstract = {There is an evident increase in the importance that remote sensing sensors play in the monitoring and evaluation of natural hazards susceptibility and risk. The present study aims to assess the flash-flood potential values, in a small catchment from Romania, using information provided remote sensing sensors and Geographic Informational Systems (GIS) databases which were involved as input data into a number of four ensemble models. In a first phase, with the help of high-resolution satellite images from the Google Earth application, 481 points affected by torrential processes were acquired, another 481 points being randomly positioned in areas without torrential processes. Seventy percent of the dataset was kept as training data, while the other 30% was assigned to validating sample. Further, in order to train the machine learning models, information regarding the 10 flash-flood predictors was extracted in the training sample locations. Finally, the following four ensembles were used to calculate the Flash-Flood Potential Index across the Bâsca Chiojdului river basin: Deep Learning Neural Network–Frequency Ratio (DLNN-FR), Deep Learning Neural Network–Weights of Evidence (DLNN-WOE), Alternating Decision Trees–Frequency Ratio (ADT-FR) and Alternating Decision Trees–Weights of Evidence (ADT-WOE). The model’s performances were assessed using several statistical metrics. Thus, in terms of Sensitivity, the highest value of 0.985 was achieved by the DLNN-FR model, meanwhile the lowest one (0.866) was assigned to ADT-FR ensemble. Moreover, the specificity analysis shows that the highest value (0.991) was attributed to DLNN-WOE algorithm, while the lowest value (0.892) was achieved by ADT-FR. During the training procedure, the models achieved overall accuracies between 0.878 (ADT-FR) and 0.985 (DLNN-WOE). K-index shows again that the most performant model was DLNN-WOE (0.97). The Flash-Flood Potential Index (FFPI) values revealed that the surfaces with high and very high flash-flood susceptibility cover between 46.57% (DLNN-FR) and 59.38% (ADT-FR) of the study zone. The use of the Receiver Operating Characteristic (ROC) curve for results validation highlights the fact that FFPIDLNN-WOE is characterized by the most precise results with an Area Under Curve of 0.96. © 2021 by the authors. Licensee MDPI, Basel, Switzerland.},
note = {21},
keywords = {},
pubstate = {published},
tppubtype = {article}
}
Pham, Q. B.; Mohammadpour, R.; Linh, N. T. T.; Mohajane, M.; Pourjasem, A.; Sammen, S. S.; Anh, D. T.; Nam, V. T.
Application of soft computing to predict water quality in wetland Journal Article
In: Environmental Science and Pollution Research, vol. 28, no. 1, pp. 185-200, 2021, ISSN: 09441344, (24).
@article{2-s2.0-85089552033,
title = {Application of soft computing to predict water quality in wetland},
author = { Q.B. Pham and R. Mohammadpour and N.T.T. Linh and M. Mohajane and A. Pourjasem and S.S. Sammen and D.T. Anh and V.T. Nam},
url = {https://www.scopus.com/inward/record.uri?eid=2-s2.0-85089552033&doi=10.1007%2fs11356-020-10344-8&partnerID=40&md5=23c6eb18d45a5697c7f46afc1e7cb5ce},
doi = {10.1007/s11356-020-10344-8},
issn = {09441344},
year = {2021},
date = {2021-01-01},
journal = {Environmental Science and Pollution Research},
volume = {28},
number = {1},
pages = {185-200},
publisher = {Springer Science and Business Media Deutschland GmbH},
abstract = {Prediction of water quality is a critical issue because of its significant impact on human and ecosystem health. This research aims to predict water quality index (WQI) for the free surface wetland using three soft computing techniques namely, adaptive neuro-fuzzy system (ANFIS), artificial neural networks (ANNs), and group method of data handling (GMDH). Seventeen wetland points for a period of 14 months were considered for monitoring water quality parameters including conductivity, suspended solid (SS), biochemical oxygen demand (BOD), ammoniacal nitrogen (AN), chemical oxygen demand (COD), dissolved oxygen (DO), temperature, pH, phosphate nitrite, and nitrate. The sensitivity analysis performed by ANFIS indicates that the significant parameters to predict WQI are pH, COD, AN, and SS. The results indicated that ANFIS with Nash-Sutcliffe Efficiency (NSE = 0.9634) and mean absolute error (MAE = 0.0219) has better performance to predict the WQI comparing with ANNs (NSE = 0.9617 and MAE = 0.0222) and GMDH (NSE = 0.9594 and MAE = 0.0245) models. However, ANNs provided a comparable prediction and the GMDH can be considered as a technique with an acceptable prediction for practical purposes. The findings of this study could be used as an effective reference for policy makers in the field of water resource management. Decreasing variables, reduction of running time, and high speed of these approaches are the most important reasons to employ them in any aquatic environment worldwide. © 2020, Springer-Verlag GmbH Germany, part of Springer Nature.},
note = {24},
keywords = {},
pubstate = {published},
tppubtype = {article}
}
2020
Salam, R.; Islam, A. R. M. T.; Pham, Q. B.; Dehghani, M.; Al-Ansari, N.; Linh, N. T. T.
The optimal alternative for quantifying reference evapotranspiration in climatic sub-regions of Bangladesh Journal Article
In: Scientific Reports, vol. 10, no. 1, 2020, ISSN: 20452322, (28).
@article{2-s2.0-85096297937,
title = {The optimal alternative for quantifying reference evapotranspiration in climatic sub-regions of Bangladesh},
author = { R. Salam and A.R.M.T. Islam and Q.B. Pham and M. Dehghani and N. Al-Ansari and N.T.T. Linh},
url = {https://www.scopus.com/inward/record.uri?eid=2-s2.0-85096297937&doi=10.1038%2fs41598-020-77183-y&partnerID=40&md5=c7464c5797f248336d2316a78f415258},
doi = {10.1038/s41598-020-77183-y},
issn = {20452322},
year = {2020},
date = {2020-01-01},
journal = {Scientific Reports},
volume = {10},
number = {1},
publisher = {Nature Research},
abstract = {Reference evapotranspiration (ETo) is a basic element for hydrological designing and agricultural water resources management. The FAO56 recommended Penman–Monteith (FAO56-PM) formula recognized worldwide as the robust and standard model for calculating ETo. However, the use of the FAO56-PM model is restricted in some data-scarce regions like Bangladesh. Therefore, it is imperative to find an optimal alternative for estimating ETo against FAO56-PM model. This study comprehensively compared the performance of 13 empirical models (Hargreaves–Samani; HargreavesM1; Hargreaves M2; Berti; WMO; Abtew; Irmak 1; Irmak 2; Makkink; Priestley-Taylor; Jensen–Haise; Tabari and Turc) by using statistical criteria for 38-years dataset from 1980 to 2017 in Bangladesh. The radiation-based model proposed by Abtew (ETo;6) was selected as an optimal alternative in all the sub-regions and whole Bangladesh against FAO56-PM model owing to its high accuracy, reliability in outlining substantial spatiotemporal variations of ETo, with very well linearly correlation with the FAO56-PM and the least errors. The importance degree analysis of 13 models based on the random forest (RF) also depicted that Abtew (ETo;6) is the most reliable and robust model for ETo computation in different sub-regions. Validation of the optimal alternative produced the largest correlation coefficient of 0.989 between ETo,s and ETo,6 and confirmed that Abtew (ETo;6) is the best suitable method for ETo calculation in Bangladesh. © 2020, The Author(s).},
note = {28},
keywords = {},
pubstate = {published},
tppubtype = {article}
}
Talukdar, S.; Ghose, B.; Shah, F.; Salam, R.; Mahato, S.; Pham, Q. B.; Linh, N. T. T.; Costache, R.; Avand, M.
Flood susceptibility modeling in Teesta River basin, Bangladesh using novel ensembles of bagging algorithms Journal Article
In: Stochastic Environmental Research and Risk Assessment, vol. 34, no. 12, pp. 2277-2300, 2020, ISSN: 14363240, (70).
@article{2-s2.0-85090216004,
title = {Flood susceptibility modeling in Teesta River basin, Bangladesh using novel ensembles of bagging algorithms},
author = { S. Talukdar and B. Ghose and F. Shah and R. Salam and S. Mahato and Q.B. Pham and N.T.T. Linh and R. Costache and M. Avand},
url = {https://www.scopus.com/inward/record.uri?eid=2-s2.0-85090216004&doi=10.1007%2fs00477-020-01862-5&partnerID=40&md5=6a99f7ac49b0ad0423c1e6c231869c95},
doi = {10.1007/s00477-020-01862-5},
issn = {14363240},
year = {2020},
date = {2020-01-01},
journal = {Stochastic Environmental Research and Risk Assessment},
volume = {34},
number = {12},
pages = {2277-2300},
publisher = {Springer Science and Business Media Deutschland GmbH},
abstract = {Abstract: The flooding in Bangladesh during monsoon season is very common and frequently happens. Consequently, people have been experiencing tremendous damage to properties, infrastructures, and human casualties. Usually, floods are one of the devastating disasters from nature, but for developing nations like Bangladesh, flooding becomes worse. Due to the dynamic and complex nature of the flooding, the prediction of flooding sites was usually very difficult for flood management. But the artificial intelligence and advanced remote sensing techniques together could predict and identify the possible sites, which are vulnerable to flooding. The present work aimed to predict and identify the flooding sites or flood susceptible zones in the Teesta River basin by employing state-of-the-art novel ensemble machine learning algorithms. We developed ensembles of bagging with REPtree, random forest (RF), M5P, and random tree (RT) algorithms for obtaining reliable and highly accurate results. Twelve factors, which are considered as the conditioning factors, and 413 current and former flooding points were identified for flooding susceptibility modelling. The Information Gain ratio statistical technique was utilized to determine the influence of the factors for flooding. We applied receiver operating characteristic curve (ROC) for validation of the flood susceptible models. The Freidman test, Wilcoxon signed-rank test, Kruskal–Wallis test and Kolmogorov–Smirnov test were applied together for the first time in flood susceptibility modelling to compare the models with each other. Results showed that more than 800 km2 area was predicted as the very high flood susceptibility zones by all algorithms. The ROC curve showed that all models achieved more than 0.85 area under the curve indicating highly accurate flood models. For flood susceptibility modelling, the bagging with M5P performed superior, followed by bagging with RF, bagging with REPtree and bagging with RT. The methodology and solution-oriented results presented in this paper will assist the regional as well as local authorities and the policy-makers for mitigating the risks related to floods and also help in developing appropriate measures to avoid potential damages. Graphic abstract: [Figure not available: see fulltext.] © 2020, Springer-Verlag GmbH Germany, part of Springer Nature.},
note = {70},
keywords = {},
pubstate = {published},
tppubtype = {article}
}
Pham, Q. B.; Afan, H. A.; Mohammadi, B.; Ahmed, A. N.; Linh, N. T. T.; Vo, N. D.; Moazenzadeh, R.; Yu, P. S.; El-Shafie, A.
Hybrid model to improve the river streamflow forecasting utilizing multi-layer perceptron-based intelligent water drop optimization algorithm Journal Article
In: Soft Computing, vol. 24, no. 23, pp. 18039-18056, 2020, ISSN: 14327643, (29).
@article{2-s2.0-85086125529,
title = {Hybrid model to improve the river streamflow forecasting utilizing multi-layer perceptron-based intelligent water drop optimization algorithm},
author = { Q.B. Pham and H.A. Afan and B. Mohammadi and A.N. Ahmed and N.T.T. Linh and N.D. Vo and R. Moazenzadeh and P.S. Yu and A. El-Shafie},
url = {https://www.scopus.com/inward/record.uri?eid=2-s2.0-85086125529&doi=10.1007%2fs00500-020-05058-5&partnerID=40&md5=3173dfff1215ff2c57ccf8505e8363ea},
doi = {10.1007/s00500-020-05058-5},
issn = {14327643},
year = {2020},
date = {2020-01-01},
journal = {Soft Computing},
volume = {24},
number = {23},
pages = {18039-18056},
publisher = {Springer Science and Business Media Deutschland GmbH},
abstract = {Artificial intelligence (AI) models have been effectively applied to predict/forecast certain variable in several engineering applications, in particular, where this variable is highly stochastic in nature and complex to identify utilizing classical mathematical model, such as river streamflow. However, the existing AI models, such as multi-layer perceptron neural network (MLP-NN), are basically incomprehensible and facing problem when applied for time series prediction or forecasting. One of the main drawbacks of the MLP-NN model is the ability of the used default optimization algorithm [gradient decent algorithm (GDA)] to search for the optimal weight and bias values associated with each neuron within the MLP-NN architecture. In fact, GDA is a first-order iteration algorithm that usually trapped in local minima, especially when the time series is highly stochastic as in the river streamflow historical records. As a result, the overall performance of the MLP-NN model experienced inaccurate prediction or forecasting for the desired output. Moreover, due to the possibility of overfitting with MLP model which may lead to poor performance of prediction of the unseen input pattern, there is need to introduce new augmented algorithm capable of identifying the complexity of streamflow data and improve the prediction accuracy. Therefore, in this study, a replacement for the GDA with advanced optimization algorithm, namely intelligent water drop (IWD), is proposed to enhance the searching procedure for the global optima. The new proposed forecasting model is, namely MLP-IWD. Two different historical rivers streamflow data have been collected from Nong Son and Thanh My stations on the Vu Gia Thu Bon river basin for period between (1978 and 2016) in order to examine the performance of the proposed MLP-IWD model. In addition, in order to evaluate the performance of the proposed MLP-IWD model under different conditions, four different scenarios for the model input–output architecture have been investigated. Results showed that the proposed MLP-IWD model outperformed the classical MLP-NN model and significantly improve the forecasting accuracy for the river streamflow. Finally, the proposed model could be generalized and applied in different rivers worldwide. © 2020, Springer-Verlag GmbH Germany, part of Springer Nature.},
note = {29},
keywords = {},
pubstate = {published},
tppubtype = {article}
}
Pham, Q. B.; Gaya, M. S.; Abba, S. I.; Abdulkadir, R. A.; Esmaili, P.; Linh, N. T. T.; Sharma, C.; Malik, A.; Khoi, D. N.; Dung, T. D.; Linh, D. Q.
Modeling of bunus regional sewage treatment plant using machine learning approaches Journal Article
In: Desalination and Water Treatment, vol. 203, pp. 80-90, 2020, ISSN: 19443994, (8).
@article{2-s2.0-85098530532,
title = {Modeling of bunus regional sewage treatment plant using machine learning approaches},
author = { Q.B. Pham and M.S. Gaya and S.I. Abba and R.A. Abdulkadir and P. Esmaili and N.T.T. Linh and C. Sharma and A. Malik and D.N. Khoi and T.D. Dung and D.Q. Linh},
url = {https://www.scopus.com/inward/record.uri?eid=2-s2.0-85098530532&doi=10.5004%2fdwt.2020.26160&partnerID=40&md5=2ffc1393c2ec63b821ca3be329875121},
doi = {10.5004/dwt.2020.26160},
issn = {19443994},
year = {2020},
date = {2020-01-01},
journal = {Desalination and Water Treatment},
volume = {203},
pages = {80-90},
publisher = {Desalination Publications},
abstract = {Certain aspects of the dynamics of wastewater treatment plants appear to be chaotic, which makes modeling of the process of wastewater treatment plants extremely difficult. An appropriate model is key for the optimal operation of the plant. Conventional prediction techniques are not good enough to produce the desired results and determination of the suitable structure of using either fuzzy, artificial neural network or adaptive neuro-fuzzy interface system becomes cum-bersome. This article proposed the application of advanced machine learning methodologies, for example, extreme learning machine (ELM), support vector machine (SVM) for modeling the Bunus regional sewage treatment plant. These advanced machine learning methods were also compared with conventional autoregressive integrated moving average (ARIMA). Observed data from the Bunus regional wastewater treatment plant was used for the modeling. The simulation results indicated that the ELM model performed better than the SVM and ARIMA models with a decrease in mean absolute percentage error by 19% and 29% than SVM and ARIMA models respectively. As the choice of input parameters often affects the modeling performance different combinations of input variables were selected. It was observed that influent biological oxygen demand, chemical oxygen demand, suspended solids, ammonium iron (NH4 ) were able to model the process better than other input parameter combinations. © 2020 Desalination Publications. All rights reserved.},
note = {8},
keywords = {},
pubstate = {published},
tppubtype = {article}
}
Abba, S. I.; Pham, Q. B.; Saini, G.; Linh, N. T. T.; Ahmed, A. N.; Mohajane, M.; Khaledian, M. R.; Abdulkadir, R. A.; Bach, Q. V.
Implementation of data intelligence models coupled with ensemble machine learning for prediction of water quality index Journal Article
In: Environmental Science and Pollution Research, vol. 27, no. 33, pp. 41524-41539, 2020, ISSN: 09441344, (38).
@article{2-s2.0-85088160474,
title = {Implementation of data intelligence models coupled with ensemble machine learning for prediction of water quality index},
author = { S.I. Abba and Q.B. Pham and G. Saini and N.T.T. Linh and A.N. Ahmed and M. Mohajane and M.R. Khaledian and R.A. Abdulkadir and Q.V. Bach},
url = {https://www.scopus.com/inward/record.uri?eid=2-s2.0-85088160474&doi=10.1007%2fs11356-020-09689-x&partnerID=40&md5=85e337a9a604c7373a4df0ba4ad58a78},
doi = {10.1007/s11356-020-09689-x},
issn = {09441344},
year = {2020},
date = {2020-01-01},
journal = {Environmental Science and Pollution Research},
volume = {27},
number = {33},
pages = {41524-41539},
publisher = {Springer Science and Business Media Deutschland GmbH},
abstract = {In recent decades, various conventional techniques have been formulated around the world to evaluate the overall water quality (WQ) at particular locations. In the present study, back propagation neural network (BPNN) and adaptive neuro-fuzzy inference system (ANFIS), support vector regression (SVR), and one multilinear regression (MLR) are considered for the prediction of water quality index (WQI) at three stations, namely Nizamuddin, Palla, and Udi (Chambal), across the Yamuna River, India. The nonlinear ensemble technique was proposed using the neural network ensemble (NNE) approach to improve the performance accuracy of the single models. The observed WQ parameters were provided by the Central Pollution Control Board (CPCB) including dissolved oxygen (DO), pH, biological oxygen demand (BOD), ammonia (NH3), temperature (T), and WQI. The performance of the models was evaluated by various statistical indices. The obtained results indicated the feasibility of the developed data intelligence models for predicting the WQI at the three stations with the superior modelling results of the NNE. The results also showed that the minimum values for root mean square (RMS) varied between 0.1213 and 0.4107, 0.003 and 0.0367, and 0.002 and 0.0272 for Nizamuddin, Palla, and Udi (Chambal), respectively. ANFIS-M3, BPNN-M4, and BPNN-M3 improved the performance with regard to an absolute error by 41%, 4%, and 3%, over other models for Nizamuddin, Palla, and Udi (Chambal) stations, respectively. The predictive comparison demonstrated that NNE proved to be effective and can therefore serve as a reliable prediction approach. The inferences of this paper would be of interest to policymakers in terms of WQ for establishing sustainable management strategies of water resources. © 2020, Springer-Verlag GmbH Germany, part of Springer Nature.},
note = {38},
keywords = {},
pubstate = {published},
tppubtype = {article}
}
Mohammed, S.; Abdo, H. G.; Szabo, S.; Pham, Q. B.; Holb, I. J.; Linh, N. T. T.; Anh, D. T.; Alsafadi, K.; Mokhtar, A.; Kbibo, I.; Ibrahim, J.; Rodrigo-Comino, J.
Estimating human impacts on soil erosion considering different hillslope inclinations and land uses in the coastal region of syria Journal Article
In: Water (Switzerland), vol. 12, no. 10, 2020, ISSN: 20734441, (19).
@article{2-s2.0-85092708721,
title = {Estimating human impacts on soil erosion considering different hillslope inclinations and land uses in the coastal region of syria},
author = { S. Mohammed and H.G. Abdo and S. Szabo and Q.B. Pham and I.J. Holb and N.T.T. Linh and D.T. Anh and K. Alsafadi and A. Mokhtar and I. Kbibo and J. Ibrahim and J. Rodrigo-Comino},
url = {https://www.scopus.com/inward/record.uri?eid=2-s2.0-85092708721&doi=10.3390%2fw12102786&partnerID=40&md5=427781b68ffd884867b96cc2ecc40e00},
doi = {10.3390/w12102786},
issn = {20734441},
year = {2020},
date = {2020-01-01},
journal = {Water (Switzerland)},
volume = {12},
number = {10},
publisher = {MDPI AG},
abstract = {Soils in the coastal region of Syria (CRoS) are one of the most fragile components of natural ecosystems. However, they are adversely affected by water erosion processes after extreme land cover modifications such as wildfires or intensive agricultural activities. The main goal of this research was to clarify the dynamic interaction between erosion processes and different ecosystem components (inclination; land cover/land use; and rainy storms) along with the vulnerable territory of the CRoS. Experiments were carried out in five different locations using a total of 15 erosion plots. Soil loss and runoff were quantified in each experimental plot, considering different inclinations and land uses (agricultural land (AG); burnt forest (BF); forest/control plot (F)). Observed runoff and soil loss varied greatly according to both inclination and land cover after 750 mm of rainfall (26 events). In the cultivated areas, the average soil water erosion ranged between 0.14 ± 0.07 and 0.74 ± 0.33 kg/m2; in the BF plots, mean soil erosion ranged between 0.03 ± 0.01 and 0.24 ± 0.10 kg/m2. The lowest amount of erosion was recorded in the F plots where the erosion ranged between 0.1 ± 0.001 and 0.07 ± 0.03 kg/m2. Interestingly, the General Linear Model revealed that all factors (i.e.; inclination; rainfall and land use) had a significant (p < 0.001) effect on the soil loss. We concluded that human activities greatly influenced soil erosion rates, being higher in the AG lands, followed by BF and F. Therefore, the current study could be very useful to policymakers and planners for proposing immediate conservation or restoration plans in a less studied area which has been shown to be vulnerable to soil erosion processes. © 2020 by the authors. Licensee MDPI, Basel, Switzerland.},
note = {19},
keywords = {},
pubstate = {published},
tppubtype = {article}
}
Kuriqi, A.; Ali, R.; Pham, Q. B.; Gambini, J. Montenegro; Gupta, V.; Malik, A.; Linh, N. T. T.; Joshi, Y.; Anh, D. T.; Nam, V. T.; Dong, X.
Seasonality shift and streamflow flow variability trends in central India Journal Article
In: Acta Geophysica, vol. 68, no. 5, pp. 1461-1475, 2020, ISSN: 18956572, (47).
@article{2-s2.0-85090310682,
title = {Seasonality shift and streamflow flow variability trends in central India},
author = { A. Kuriqi and R. Ali and Q.B. Pham and J. Montenegro Gambini and V. Gupta and A. Malik and N.T.T. Linh and Y. Joshi and D.T. Anh and V.T. Nam and X. Dong},
url = {https://www.scopus.com/inward/record.uri?eid=2-s2.0-85090310682&doi=10.1007%2fs11600-020-00475-4&partnerID=40&md5=491f6cc2647c9d6cdf17e0a7d40a3bb5},
doi = {10.1007/s11600-020-00475-4},
issn = {18956572},
year = {2020},
date = {2020-01-01},
journal = {Acta Geophysica},
volume = {68},
number = {5},
pages = {1461-1475},
publisher = {Springer Science and Business Media Deutschland GmbH},
abstract = {A better understanding of intra/inter-annual streamflow variability and trends enables more effective water resources planning and management for current and future needs. This paper investigates the variability and trends of streamflow data from five stations (i.e. Ashti; Chindnar; Pathgudem; Polavaram; and Tekra) in Godavari river basin, India. The streamflow data were obtained from the Indian Central Water Commission and cover more than 30 years of mean daily records (i.e. 1972–2011). The streamflow data were statistically assessed using Gamma, Generalised Extreme Value and Normal distributions to understand the probability distribution features of data at inter-annual time-scale. Quantifiable changes in observed streamflow data were identified by Sen’s slope method. Two other nonparametric, Mann–Kendall and Innovative Trend Analysis methods were also applied to validate findings from Sen’s slope trend analysis. The mean flow discharge for each month (i.e. January to December), seasonal variation (i.e. Spring; Summer; Autumn; and Winter) as well as an annual mean, annual maximum and minimum flows were analysed for each station. The results show that three stations (i.e. Ashti; Tekra; and Polavaram) demonstrate an increasing trend, notably during Winter and Spring. In contrast, two other stations (i.e. Pathgudem; Chindnar) revealed a decreasing trend almost at all seasons. A significant decreasing trend was observed at all station over Summer and Autumn seasons. Notably, all stations showed a decreasing trend in maximum flows; remarkably, Tekra station revealed the highest decreasing magnitude. Significant decrease in minimum flows was observed in two stations only, Chindnar and Pathgudem. Findings resulted from this study might be useful for water managers and decision-makers to propose more sustainable water management recommendations and practices. © 2020, Institute of Geophysics, Polish Academy of Sciences & Polish Academy of Sciences.},
note = {47},
keywords = {},
pubstate = {published},
tppubtype = {article}
}
Guan, Y.; Mohammadi, B.; Pham, Q. B.; Adarsh, S.; Balkhair, K. S.; Rahman, K. U.; Linh, N. T. T.; Tri, D. Q.
In: Theoretical and Applied Climatology, vol. 142, no. 1-2, pp. 349-367, 2020, ISSN: 0177798X, (20).
@article{2-s2.0-85087815102,
title = {A novel approach for predicting daily pan evaporation in the coastal regions of Iran using support vector regression coupled with krill herd algorithm model},
author = { Y. Guan and B. Mohammadi and Q.B. Pham and S. Adarsh and K.S. Balkhair and K.U. Rahman and N.T.T. Linh and D.Q. Tri},
url = {https://www.scopus.com/inward/record.uri?eid=2-s2.0-85087815102&doi=10.1007%2fs00704-020-03283-4&partnerID=40&md5=c841cc6e50cac7d5814191921b491119},
doi = {10.1007/s00704-020-03283-4},
issn = {0177798X},
year = {2020},
date = {2020-01-01},
journal = {Theoretical and Applied Climatology},
volume = {142},
number = {1-2},
pages = {349-367},
publisher = {Springer},
abstract = {Evaporation is one of the vital components of hydrological cycle. Precise estimation of pan evaporation (Epan) is essential for the sustainable water resources management. The current study proposed a novel approach to estimate daily Epan across the humid region of Iran using support vector regression (SVR) technique coupled with Krill Herd Algorithm (SVR-KHA). Meteorological data were collected from three stations (Bandar Abbas; Rudsar; and Osku) over a period from 2008 to 2018 and used for application. Of the data, 70% were used for training and remaining 30% were used for testing. The study considered seven different combinations of input variables for predicting daily Epan at each station. The influence of KHA hybridization is examined by comparing results of SVR-KHA algorithm with simple SVR through a multitude of statistical performance evaluation criteria such as coefficient of determination (R2), Wilmot’s index (WI), root-mean-square error (RMSE), Mean Absolute Error (MAE), Relative Root Mean Square Error (RRMSE), Mean Absolute Relative Error (MARE), and several graphical tools. Single input SVR1 model hybrid with KHA (SVR-KHA1) showed improved performance (R2 of 0.717 and RMSE of 1.032 mm/day) as compared with multi-input SVR models, e.g., SVR5 (with RMSE and MAE of 1.037 mm/day and 0.773 mm/day), while SVR7 model hybridized with KHA (SVR-KHA7), which considers seven meteorological variables as input, performed best as compared with other models considered in this study. Epan estimates at Bandar Abbas and Rudsar by SVR and SVR-KHA are similar (with R2 statistics values of 0.82 and 0.84 at Bandar Abbas station; and 0.88 and 0.9 at Rudsar station; respectively). However, better improvements in Epan estimates are observed at Osku station (with R2 of 0.91 and 0.86; respectively), which is situated at interior geographical location with a higher altitude than the other two coastal stations. Overall, the results showed consistent performance of SVR-KHA model with stable residuals of lower magnitude as compared with standalone SVR models. © 2020, Springer-Verlag GmbH Austria, part of Springer Nature.},
note = {20},
keywords = {},
pubstate = {published},
tppubtype = {article}
}
Ali, S. A.; Parvin, F.; Pham, Q. B.; Vojtek, M.; Vojteková, J.; Costache, R.; Linh, N. T. T.; Nguyen, H. Q.; Ahmad, A.; Ghorbani, M. A.
In: Ecological Indicators, vol. 117, 2020, ISSN: 1470160X, (82).
@article{2-s2.0-85086627928,
title = {GIS-based comparative assessment of flood susceptibility mapping using hybrid multi-criteria decision-making approach, naïve Bayes tree, bivariate statistics and logistic regression: A case of Topľa basin, Slovakia},
author = { S.A. Ali and F. Parvin and Q.B. Pham and M. Vojtek and J. Vojteková and R. Costache and N.T.T. Linh and H.Q. Nguyen and A. Ahmad and M.A. Ghorbani},
url = {https://www.scopus.com/inward/record.uri?eid=2-s2.0-85086627928&doi=10.1016%2fj.ecolind.2020.106620&partnerID=40&md5=79d4cf47c90f0fa953cf6a8125f35802},
doi = {10.1016/j.ecolind.2020.106620},
issn = {1470160X},
year = {2020},
date = {2020-01-01},
journal = {Ecological Indicators},
volume = {117},
publisher = {Elsevier B.V.},
abstract = {Flood is a devastating natural hazard that may cause damage to the environment infrastructure, and society. Hence, identifying the susceptible areas to flood is an important task for every country to prevent such dangerous consequences. The present study developed a framework for identifying flood-prone areas of the Topľa river basin, Slovakia using geographic information system (GIS), multi-criteria decision making approach (MCDMA), bivariate statistics (Frequency Ratio (FR); Statistical Index (SI)) and machine learning (Naïve Bayes Tree (NBT); Logistic Regression (LR)). To reach such a goal, different physical-geographical factors (criteria) were integrated and mapped. To access the relationship and interdependences among the criteria, decision-making trial and evaluation laboratory (DEMATEL) and analytic network process (ANP) were used. Based on the experts’ decisions, the DEMATEL-ANP model was used to compute the relative weights of different criteria and a GIS-based linear combination was performed to derive the susceptibility index. Separately, the flood susceptibility index computation through NBT-FR and NBT-SI hybrid models assumed, in the first stage, the estimation of the weight of each class/category of conditioning factor through SI and FR and the integration of these values in NBT algorithm. The application of LR stand-alone required the calculation of the weights of conditioning factors by analysing their spatial relation with the location of the historical flood events. The study revealed that very high and high flood susceptibility classes covered between 20% and 47% of the study area, respectively. The validation of results, using the past flood points, highlighted that the hybrid DEMATEL-ANP model was the most performant with an Area Under ROC curve higher than 0.97, an accuracy of 0.922 and a value of HSS of 0.844. The presented methodological approach used for the identification of flood susceptible areas can serve as an alternative for the updating of preliminary flood risk assessment based on the EU Floods Directive. © 2020 Elsevier Ltd},
note = {82},
keywords = {},
pubstate = {published},
tppubtype = {article}
}
Mohammed, S.; Al-Ebraheem, A.; Holb, I. J.; Alsafadi, K.; Dikkeh, M.; Pham, Q. B.; Linh, N. T. T.; Szabo, S.
In: Water (Switzerland), vol. 12, no. 9, 2020, ISSN: 20734441, (22).
@article{2-s2.0-85090981264,
title = {Soil management effects on soil water erosion and runoff in Central Syria-A comparative evaluation of general linear model and random forest regression},
author = { S. Mohammed and A. Al-Ebraheem and I.J. Holb and K. Alsafadi and M. Dikkeh and Q.B. Pham and N.T.T. Linh and S. Szabo},
url = {https://www.scopus.com/inward/record.uri?eid=2-s2.0-85090981264&doi=10.3390%2fw12092529&partnerID=40&md5=a5e2454a6d0deb96f9c96dab5e54b1e2},
doi = {10.3390/w12092529},
issn = {20734441},
year = {2020},
date = {2020-01-01},
journal = {Water (Switzerland)},
volume = {12},
number = {9},
publisher = {MDPI AG},
abstract = {The Mediterranean part of Syria is affected by soil water erosion due to poor land management. Within this context, the main aim of this research was to track soil erosion and runoff after each rainy storm between September 2013 and April 2014 (rainy season), on two slopes with different gradients (4.7%; 10.3%), under three soil cover types (SCTs): bare soil (BS), metal sieve cover (MC), and strip cropping (SC), in Central Syria. Two statistical multivariate models, the general linear model (GLM), and the random forest regression (RFR) were applied to reveal the importance of SCTs. Our results reveal that higher erosion rate, as well as runoff, were recorded in BS followed by MC, and SC. Accordingly, soil cover had a significant effect (p < 0.001) on soil erosion, and no significant difference was detected between MC and SC. Different combinations of slopes and soil cover had no effect on erosion, at least in this experiment. RFR performed better than GLM in predictions. GLM's median of mean absolute error was 21% worse than RFR. Nonetheless, 25 repetitions of 2-fold cross-validation ensured the highest available prediction accuracy for RFR. In conclusion, we revealed that runoff, rain intensity and soil cover were the most important factors in erosion. © 2020 by the authors.},
note = {22},
keywords = {},
pubstate = {published},
tppubtype = {article}
}
Malik, A.; Kumar, A. Ruban; Pham, Q. B.; Zhu, S.; Linh, N. T. T.; Tri, D. Q.
Identification of EDI trend using Mann-Kendall and Şen-Innovative Trend methods (Uttarakhand, India) Journal Article
In: Arabian Journal of Geosciences, vol. 13, no. 18, 2020, ISSN: 18667511, (9).
@article{2-s2.0-85090896446,
title = {Identification of EDI trend using Mann-Kendall and Şen-Innovative Trend methods (Uttarakhand, India)},
author = { A. Malik and A. Ruban Kumar and Q.B. Pham and S. Zhu and N.T.T. Linh and D.Q. Tri},
url = {https://www.scopus.com/inward/record.uri?eid=2-s2.0-85090896446&doi=10.1007%2fs12517-020-05926-2&partnerID=40&md5=17769f83f504f6c54e58294f5bb51c71},
doi = {10.1007/s12517-020-05926-2},
issn = {18667511},
year = {2020},
date = {2020-01-01},
journal = {Arabian Journal of Geosciences},
volume = {13},
number = {18},
publisher = {Springer Science and Business Media Deutschland GmbH},
abstract = {Under the climate change scenario, the identification of drought trends is primarily essential for the efficient utilization of water resources. In this paper, two methods, including traditional Mann-Kendall (MK) and graphical Şen-Innovative Trend (ŞIT), were utilized for Effective Drought Index (EDI) trend detection at 13 meteorological stations situated in the State of Uttarakhand, India. The EDI was computed for 54 years from 1962 to 2015 using monthly rainfall data at the study stations. The magnitude (mm/year) of the EDI was derived by Sen’s-Slope Estimator (SSE) method. In total, 156 series of data were analyzed, and the results showed that the ŞIT method detected a significantly negative/positive trend in 71/60 time series, while the MK method detected a significantly negative/positive trend in 25/9 time series from January to December at the study stations. Magnitude (mm/year) varies from − 0.0275 to 0.0256 (January), − 0.0352 to 0.0343 (February), − 0.0312 to 0.0312 (March), − 0.0343 to 0.0276 (April), − 0.0359 to 0.0237 (May), − 0.0293 to 0.0205 (June), − 0.0234 to 0.0235 (July), − 0.0277 to 0.0405 (August), − 0.0297 to 0.0247 (September), − 0.0288 to 0.0227 (October), − 0.0290 to 0.0241 (November), and − 0.0298 to 0.0236 (December) over the study region. Additionally, the results indicated the supremacy of the ŞIT method by examining the unobserved trend that cannot be detected by the MK method over the study region in the EDI data series. In general, the EDI trend was found negative (decreasing) and positive (increasing), which suggested that more attention should be paid towards drought and wet (i.e.; moderate; severe; extreme) at the study stations. The results of this research can be employed for water resource management and understanding the characteristics of climate variation over the study area. © 2020, Saudi Society for Geosciences.},
note = {9},
keywords = {},
pubstate = {published},
tppubtype = {article}
}
Mohammadi, B.; Ahmadi, F.; Mehdizadeh, S.; Guan, Y.; Pham, Q. B.; Linh, N. T. T.; Tri, D. Q.
Developing Novel Robust Models to Improve the Accuracy of Daily Streamflow Modeling Journal Article
In: Water Resources Management, vol. 34, no. 10, pp. 3387-3409, 2020, ISSN: 09204741, (41).
@article{2-s2.0-85087883264,
title = {Developing Novel Robust Models to Improve the Accuracy of Daily Streamflow Modeling},
author = { B. Mohammadi and F. Ahmadi and S. Mehdizadeh and Y. Guan and Q.B. Pham and N.T.T. Linh and D.Q. Tri},
url = {https://www.scopus.com/inward/record.uri?eid=2-s2.0-85087883264&doi=10.1007%2fs11269-020-02619-z&partnerID=40&md5=3862a129d480721bbacbbe676c7c0385},
doi = {10.1007/s11269-020-02619-z},
issn = {09204741},
year = {2020},
date = {2020-01-01},
journal = {Water Resources Management},
volume = {34},
number = {10},
pages = {3387-3409},
publisher = {Springer},
abstract = {Streamflow plays a major role in the optimal management and allocation of available water resources in each region. Reliable techniques are therefore needed to be developed for streamflow modeling. In the present study, the performance of streamflow modeling is improved via developing novel boosted models. The daily streamflows of four hydrometric stations comprising of the Brantford and Galt stations located on the Grand River, Canada, as well as Macon and Elkton stations respectively, located on the Ocmulgee and Umpqua rivers, United States, are used. Three different types of boosted models are implemented and proposed by coupling the classical multi-layer perceptron (MLP) with the optimization algorithms, including particle swarm optimization (PSO) and coupled particle swarm optimization-multi-verse optimizer (PSOMVO) and a time series model, namely the bi-linear (BL). So, the boosted MLP-PSO, MLP-PSOMVO, and MLP-BL models are developed. The accuracy of all the boosted models is compared with the classical MLP and BL by the statistical metrics used. It is concluded that all the boosted models developed at the studied stations lead to superior modeling results of the daily streamflows to the classical MLP; however, the boosted MLP-BL models generally outperformed the MLP-PSO and MLP-PSOMVO ones. © 2020, Springer Nature B.V.},
note = {41},
keywords = {},
pubstate = {published},
tppubtype = {article}
}
Mohammadi, B.; Linh, N. T. T.; Pham, Q. B.; Ahmed, A. N.; Vojteková, J.; Guan, Y.; Abba, S. I.; El-Shafie, A.
Adaptive neuro-fuzzy inference system coupled with shuffled frog leaping algorithm for predicting river streamflow time series Journal Article
In: Hydrological Sciences Journal, vol. 65, no. 10, pp. 1738-1751, 2020, ISSN: 02626667, (55).
@article{2-s2.0-85086121489,
title = {Adaptive neuro-fuzzy inference system coupled with shuffled frog leaping algorithm for predicting river streamflow time series},
author = { B. Mohammadi and N.T.T. Linh and Q.B. Pham and A.N. Ahmed and J. Vojteková and Y. Guan and S.I. Abba and A. El-Shafie},
url = {https://www.scopus.com/inward/record.uri?eid=2-s2.0-85086121489&doi=10.1080%2f02626667.2020.1758703&partnerID=40&md5=cf07bbec0762557a252eef99332bc89c},
doi = {10.1080/02626667.2020.1758703},
issn = {02626667},
year = {2020},
date = {2020-01-01},
journal = {Hydrological Sciences Journal},
volume = {65},
number = {10},
pages = {1738-1751},
publisher = {Taylor and Francis Ltd.},
abstract = {Accurate runoff forecasting plays a key role in catchment water management and water resources system planning. To improve the prediction accuracy, one needs to strive to develop a reliable and accurate forecasting model for streamflow. In this study, the novel combination of the adaptive neuro-fuzzy inference system (ANFIS) model with the shuffled frog-leaping algorithm (SFLA) is proposed. Historical streamflow data of two different rivers were collected to examine the performance of the proposed model. To evaluate the performance of the proposed ANFIS-SFLA model, six different scenarios for the model input–output architecture were investigated. The results show that the proposed ANFIS-SFLA model (R2 = 0.88; NS = 0.88; RMSE = 142.30 (m3/s); MAE = 88.94 (m3/s); MAPE = 35.19%) significantly improved the forecasting accuracy and outperformed the classic ANFIS model (R2 = 0.83; NS = 0.83; RMSE = 167.81; MAE = 115.83 (m3/s); MAPE = 45.97%). The proposed model could be generalized and applied in different rivers worldwide. © 2020 IAHS.},
note = {55},
keywords = {},
pubstate = {published},
tppubtype = {article}
}
Costache, R.; Pham, Q. B.; Avand, M.; Linh, N. T. T.; Vojtek, M.; Vojteková, J.; Lee, S.; Khoi, D. N.; Nhi, P. T. Thao; Dung, T. D.
Novel hybrid models between bivariate statistics, artificial neural networks and boosting algorithms for flood susceptibility assessment Journal Article
In: Journal of Environmental Management, vol. 265, 2020, ISSN: 03014797, (53).
@article{2-s2.0-85083675804,
title = {Novel hybrid models between bivariate statistics, artificial neural networks and boosting algorithms for flood susceptibility assessment},
author = { R. Costache and Q.B. Pham and M. Avand and N.T.T. Linh and M. Vojtek and J. Vojteková and S. Lee and D.N. Khoi and P.T. Thao Nhi and T.D. Dung},
url = {https://www.scopus.com/inward/record.uri?eid=2-s2.0-85083675804&doi=10.1016%2fj.jenvman.2020.110485&partnerID=40&md5=3a3307334f3d1290fb4d02da8cce142a},
doi = {10.1016/j.jenvman.2020.110485},
issn = {03014797},
year = {2020},
date = {2020-01-01},
journal = {Journal of Environmental Management},
volume = {265},
publisher = {Academic Press},
abstract = {Across the world, the flood magnitude is expected to increase as well as the damage caused by their occurrence. In this case, the prediction of areas which are highly susceptible to these phenomena becomes very important for the authorities. The present study is focused on the evaluation of flood potential within Trotuș river basin in Romania using six ensemble models created by the combination of Analytical Hierarchy Process (AHP), Certainty Factor (CF) and Weights of Evidence (WOE) on one hand, and Gradient Boosting Trees (GBT) and Multilayer Perceptron (MLP) on the other hand. A number of 12 flood predictors, 172 flood locations and 172 non-flood locations were used. A percentage of 70% of flood and non-flood locations were used as input in models. From the input data, 70% were used as training sample and 30% as validating sample. The highest accuracy was obtained by the MLP-CF model in terms of both training (0.899) and testing (0.889) samples. A percentage between 21.88% and 36.33% of study area is covered with high and very high flood potential. The results validation, performed through the ROC Curve method, highlights that the MLP-CF model provided the most accurate results. © 2020 Elsevier Ltd},
note = {53},
keywords = {},
pubstate = {published},
tppubtype = {article}
}
Costache, R.; Pham, Q. B.; Corodescu-Roşca, E.; Cîmpianu, C.; Hong, H.; Linh, N. T. T.; Fai, C. M.; Ahmed, A. N.; Vojtek, M.; Pandhiani, S. M.; Minea, G.; Ciobotaru, N.; Popa, M. C.; Diaconu, D. C.; Pham, Bi. T.
Using GIS, remote sensing, and machine learning to highlight the correlation between the land-use/land-cover changes and flash-flood potential Journal Article
In: Remote Sensing, vol. 12, no. 9, 2020, ISSN: 20724292, (26).
@article{2-s2.0-85085504419,
title = {Using GIS, remote sensing, and machine learning to highlight the correlation between the land-use/land-cover changes and flash-flood potential},
author = { R. Costache and Q.B. Pham and E. Corodescu-Roşca and C. Cîmpianu and H. Hong and N.T.T. Linh and C.M. Fai and A.N. Ahmed and M. Vojtek and S.M. Pandhiani and G. Minea and N. Ciobotaru and M.C. Popa and D.C. Diaconu and Bi.T. Pham},
url = {https://www.scopus.com/inward/record.uri?eid=2-s2.0-85085504419&doi=10.3390%2fRS12091422&partnerID=40&md5=998ff6628f56ed2d1b8d105ea9480516},
doi = {10.3390/RS12091422},
issn = {20724292},
year = {2020},
date = {2020-01-01},
journal = {Remote Sensing},
volume = {12},
number = {9},
publisher = {MDPI AG},
abstract = {The aim of the present study was to explore the correlation between the land-use/land cover change and the flash-flood potential changes in Zabala catchment (Romania) between 1989 and 2019. In this regard, the efficiency of GIS, remote sensing and machine learning techniques in detecting spatial patterns of the relationship between the two variables was tested. The paper elaborated upon an answer to the increase in flash flooding frequency across the study area and across the earth due to the occurred land-use/land-cover changes, as well as due to the present climate change, which determined the multiplication of extreme meteorological phenomena. In order to reach the above-mentioned purpose, two land-uses/land-covers (for 1989 and 2019) were obtained using Landsat image processing and were included in a relative evolution indicator (total relative difference-synthetic dynamic land-use index), aggregated at a grid-cell level of 1 km2. The assessment of runoff potential was made with a multilayer perceptron (MLP) neural network, which was trained for 1989 and 2019 with the help of 10 flash-flood predictors, 127 flash-flood locations, and 127 non-flash-flood locations. For the year 1989, the high and very high surface runoff potential covered around 34% of the study area, while for 2019, the same values accounted for approximately 46%. The MLP models performed very well, the area under curve (AUC) values being higher than 0.837. Finally, the land-use/land-cover change indicator, as well as the relative evolution of the flash flood potential index, was included in a geographically weighted regression (GWR). The results of the GWR highlights that high values of the Pearson coefficient (r) occupied around 17.4% of the study area. Therefore, in these areas of the Zabala river catchment, the land-use/land-cover changes were highly correlated with the changes that occurred in flash-flood potential. © 2020 by the authors.},
note = {26},
keywords = {},
pubstate = {published},
tppubtype = {article}
}
Abba, S. I.; Pham, Q. B.; Usman, A. G.; Linh, N. T. T.; Aliyu, D. S.; Nguyen, Q.; Bach, Q. V.
Emerging evolutionary algorithm integrated with kernel principal component analysis for modeling the performance of a water treatment plant Journal Article
In: Journal of Water Process Engineering, vol. 33, 2020, ISSN: 22147144, (42).
@article{2-s2.0-85076541959,
title = {Emerging evolutionary algorithm integrated with kernel principal component analysis for modeling the performance of a water treatment plant},
author = { S.I. Abba and Q.B. Pham and A.G. Usman and N.T.T. Linh and D.S. Aliyu and Q. Nguyen and Q.V. Bach},
url = {https://www.scopus.com/inward/record.uri?eid=2-s2.0-85076541959&doi=10.1016%2fj.jwpe.2019.101081&partnerID=40&md5=0c62fde5c723a60c91d31904741ce458},
doi = {10.1016/j.jwpe.2019.101081},
issn = {22147144},
year = {2020},
date = {2020-01-01},
journal = {Journal of Water Process Engineering},
volume = {33},
publisher = {Elsevier Ltd},
abstract = {Providing a robust and reliable model is essential for hydro-environmental and public health engineering perspectives, including water treatment plants (WTPs). The current research develops an emerging evolutionary data-intelligence model: extreme learning machine (ELM) integrated with kernel principal component analysis (KPCA) to predict the performance of the Tamburawa WTP in Kano, Nigeria. A traditional feed-forward neural network (FFNN) and a classical linear autoregressive (AR) models were also employed to compare the predictive performance. For this purpose, different input data with the corresponding treated pH, turbidity, total dissolve solids, and hardness as the target variables obtained from the WTP were used. The predictive models are evaluated based on the three numerical indices, namely Nash-Sutcliffe (NC), root mean squared error (RMSE) and mean absolute percentage error (MAPE). To examine the similarities and differences between the observed and predicted values, a two-dimension graphical diagram (i.e.; Taylor diagram) was also utilized. The predictive results revealed the potential of KPCA-ELM, which exhibited a high level of accuracy in comparison to the single models for all the considered variables with a slight exception in terms of pH prediction. Two different model combination were built for each single (FFNN; ELM; and AR) model and KPCA algorithms (KPCA-FFNN; KPCA-ELM; and KPCA-AR). The results also depicted that both ELM and FFNN models demonstrated prediction skill and therefore, can serve as reliable models. The outcomes may contribute to the aforementioned modeling of the treated parameters and provides a reference benchmark for wastewater management and control in the Tamburawa WTP. © 2019 Elsevier Ltd},
note = {42},
keywords = {},
pubstate = {published},
tppubtype = {article}
}
Pham, Q. B.; Mukherjee, K.; Norouzi, A.; Linh, N. T. T.; Janizadeh, S.; Ahmadi, K.; Cerdà, A.; Doan, T. N. C.; Anh, D. T.
Head-cut gully erosion susceptibility modelling based on ensemble Random Forest with oblique decision trees in Fareghan watershed, Iran Journal Article
In: Geomatics, Natural Hazards and Risk, vol. 11, no. 1, pp. 2385-2410, 2020, ISSN: 19475705, (8).
@article{2-s2.0-85095837285,
title = {Head-cut gully erosion susceptibility modelling based on ensemble Random Forest with oblique decision trees in Fareghan watershed, Iran},
author = { Q.B. Pham and K. Mukherjee and A. Norouzi and N.T.T. Linh and S. Janizadeh and K. Ahmadi and A. Cerdà and T.N.C. Doan and D.T. Anh},
url = {https://www.scopus.com/inward/record.uri?eid=2-s2.0-85095837285&doi=10.1080%2f19475705.2020.1837968&partnerID=40&md5=749be5e58106337ecaa64da216d0b879},
doi = {10.1080/19475705.2020.1837968},
issn = {19475705},
year = {2020},
date = {2020-01-01},
journal = {Geomatics, Natural Hazards and Risk},
volume = {11},
number = {1},
pages = {2385-2410},
publisher = {Taylor and Francis Ltd.},
abstract = {Gully erosion is the most active hydro-geomorphological phenomenon in the continental areas due to the high erosion rates triggered by the gully system. Monitoring and modelling gully development and gully distribution will contribute to understand landforms evolution and risk assessment. The purpose of the current research is to model head-cut gully erosion susceptibility (HCGES) using support vector machine (SVM), random forest (RF) and novel ensemble model of random forest with four Oblique methods (Logistic Regression; Ridge Regression; Partial least squares (PLS) and Support vector machine (SVM)) (hereafter called ensemble ORF) data mining models in Fareghan watershed, Hormozghan province, Iran. For this purpose, 14 variables influencing the gully development, were prepared and 145 head-cut gully erosion locations were identified in the study area. The efficiency of SVM, RF, ensemble ORF were evaluated based on receiver operating characteristic (ROC), the results have shown that all these three models are highly accurate and robust in predicting the head-cut gully erosion susceptibility zones. The results of the models were evaluated based on the area under the receiver operatic characteristic curve (AUC) in the validation stage presented that the efficiency of these models are 0.91, 0.94, and 0.96, respectively. Altitude and distance from the road in all three models were more important than other variables. The findings of this research will contribute to develop gully control strategies and to prevent the gully initiation where gully erosion is more susceptible. © 2020 The Author(s). Published by Informa UK Limited, trading as Taylor & Francis Group.},
note = {8},
keywords = {},
pubstate = {published},
tppubtype = {article}
}
Abba, S. I.; Linh, N. T. T.; Abdullahi, J.; Ali, S. I. A.; Pham, Q. B.; Abdulkadir, R. A.; Costache, R.; Nam, V. T.; Anh, D. T.
Hybrid machine learning ensemble techniques for modeling dissolved oxygen concentration Journal Article
In: IEEE Access, vol. 8, pp. 157218-157237, 2020, ISSN: 21693536, (22).
@article{2-s2.0-85091213249,
title = {Hybrid machine learning ensemble techniques for modeling dissolved oxygen concentration},
author = { S.I. Abba and N.T.T. Linh and J. Abdullahi and S.I.A. Ali and Q.B. Pham and R.A. Abdulkadir and R. Costache and V.T. Nam and D.T. Anh},
url = {https://www.scopus.com/inward/record.uri?eid=2-s2.0-85091213249&doi=10.1109%2fACCESS.2020.3017743&partnerID=40&md5=db654265866e1afbfbb00f70f8f449ef},
doi = {10.1109/ACCESS.2020.3017743},
issn = {21693536},
year = {2020},
date = {2020-01-01},
journal = {IEEE Access},
volume = {8},
pages = {157218-157237},
publisher = {Institute of Electrical and Electronics Engineers Inc.},
abstract = {The reliable prediction of dissolved oxygen concentration (DO) is significantly crucial for protecting the health of the aquatic ecosystem. The current research employed four different single AI-based models, namely long short-term memory neural network (LSTM), extreme learning machine (ELM), Hammerstein-Weiner (HW) and general regression neural network (GRNN) for modeling the DO concentration of Kinta River, Malaysia using available water quality (WQ) parameters. Afterwards, the first scenario used four different ensemble techniques (ET). Two linear, i.e. simple averaging ensemble (SAE) and weighted averaging ensemble (WAE) and two nonlinear namely; backpropagation neural network ensemble (BPNN-E) and HW ensemble (HW-E). The second scenario employed a hybrid random forest (RF) ensemble in order to enhance the prediction accuracy of the single models. The WQ parameters were subjected to a different pre-analysis test to ascertain their stability. The four-model combinations are generated using the nonlinear sensitivity input selection approach. The modeling performance was assessed using the statistical measures of Nash-Sutcliffe coefficient efficiency (NSE), Willmott's index of agreement (WI), root mean square error (RMSE), mean absolute error (MAE) and mean square error (MSE) and correlation coefficient (CC). The results of the single AI-based models demonstrated that HW (M3) served as the best model for predicting DO concentration. For ensemble results, BPNN-E (WI = 0.9764) was superior to the other three ET with average decreased of more than 2% with regards to MAE. Investigation on the hybrid RF ensemble demonstrated the reliable accuracy for all the hybrid models with better predictive skill shown by the HW-RF (CC = 0.981) ensemble. The overall results verified the promising impact of HW-M3, ET and hybrid RF ensemble for the prediction of the DO concentration in the Kinta River, Malaysia. © 2013 IEEE.},
note = {22},
keywords = {},
pubstate = {published},
tppubtype = {article}
}
Mohammed, S.; Alsafadi, K.; Ali, H.; Mousavi, S. M. N.; Kiwan, S.; Hennawi, S.; Harsanyie, E.; Pham, Q. B.; Linh, N. T. T.; Ali, R.; Anh, D. T.; Thai, V. N.
In: Geocarto International, pp. 1-19, 2020, ISSN: 10106049, (12).
@article{2-s2.0-85088144489,
title = {Assessment of land suitability potentials for winter wheat cultivation by using a multi criteria decision Support- Geographic information system (MCDS-GIS) approach in Al-Yarmouk Basin (S syria)},
author = { S. Mohammed and K. Alsafadi and H. Ali and S.M.N. Mousavi and S. Kiwan and S. Hennawi and E. Harsanyie and Q.B. Pham and N.T.T. Linh and R. Ali and D.T. Anh and V.N. Thai},
url = {https://www.scopus.com/inward/record.uri?eid=2-s2.0-85088144489&doi=10.1080%2f10106049.2020.1790674&partnerID=40&md5=b1b55e426bdcd20c2d8266daf02e7147},
doi = {10.1080/10106049.2020.1790674},
issn = {10106049},
year = {2020},
date = {2020-01-01},
journal = {Geocarto International},
pages = {1-19},
publisher = {Taylor and Francis Ltd.},
abstract = {In the last few years, the agricultural sector in Syria has suffered from major problems related to land degradation. To cope with this problem, a land suitability assessment has become an essential tool for sustainable land use management. The present research qualitatively evaluated the suitability of land in the Al-Yarmouk Basin (S-Syria) for rainfed winter wheat (Triticum aestivum) cultivation. In this study, a regional spatial approach involving three steps was developed, based on the method proposed by Sys et al. In the first step, a soil survey was carried out and 107 soil profiles were described, sampled and analyzed. In the second step, climatic gridded datasets from 1984–2014 MRm at a high spatial resolution (30 meters) and the Digital Elevation Model (DEM) were clipped from NASA's Shuttle Radar Topography Mission (SRTM) and prepared for the study area. In the third step, a land suitability assessment was performed using the geographical information system (GIS) and multi criteria decision support (MCDS). Soil survey outcomes showed that the study area was dominated by five soil orders: Mollisols, Inceptisols, Vertisols, Entisols and Aridisols. Also, results from the Sys model illustrated that more than 23.8% of the study area is highly suitable (S1–0) for wheat production without any limitations, whereas 38.7% and 37.5% are highly suitable (S1–1) and moderately suitable (S2), respectively. Also, the study emphasizes the important role of topographical factors in the study area for wheat cultivation. All in all, this research suggests W-Syria as a potential region for wheat cultivation, instead of the eastern area which is subject to climate change and a shortage of water. Integrating the Sys-approach and the GIS framework offers a good tool for policy-makers to apply in Syria for land suitability assessments. © 2020, © 2020 Informa UK Limited, trading as Taylor & Francis Group.},
note = {12},
keywords = {},
pubstate = {published},
tppubtype = {article}
}
Fayaz, M.; Pham, Q. B.; Linh, N. T. T.; Nhi, P. T. T.; Khoi, D. N.; Qureshi, M. S.; Shah, A. S.; Khalid, S.
A water supply pipeline risk analysis methodology based on DIY and hierarchical fuzzy inference Journal Article
In: Symmetry, vol. 12, no. 1, 2020, ISSN: 20738994, (4).
@article{2-s2.0-85079616377,
title = {A water supply pipeline risk analysis methodology based on DIY and hierarchical fuzzy inference},
author = { M. Fayaz and Q.B. Pham and N.T.T. Linh and P.T.T. Nhi and D.N. Khoi and M.S. Qureshi and A.S. Shah and S. Khalid},
url = {https://www.scopus.com/inward/record.uri?eid=2-s2.0-85079616377&doi=10.3390%2fSYM12010044&partnerID=40&md5=eb353002eca54ba6eab974e2d111f418},
doi = {10.3390/SYM12010044},
issn = {20738994},
year = {2020},
date = {2020-01-01},
journal = {Symmetry},
volume = {12},
number = {1},
publisher = {MDPI AG},
abstract = {The standard manufacturing organizations follow certain rules. The highest ubiquitous organizing principles in infrastructure design are modular idea and symmetry, both of which are of the utmost importance. Symmetry is a substantial principle in the manufacturing industry. Symmetrical procedures act as the structural apparatus for manufacturing design. The rapid growth of population needs outstrip infrastructure such as roads, bridges, railway lines, commercial, residential buildings, etc. Numerous underground facilities are also installed to fulfill different requirements of the people. In these facilities one of the most important facility is water supply pipelines. Therefore, it is essential to regularly analyze the water supply pipelines' risk index in order to escape from economic and human losses. In this paper, we proposed a simplified hierarchical fuzzy logic (SHFL) model to reduce the set of rules. To this end, we have considered four essential factors of water supply pipelines as input to the proposed SHFL model that are: leakage, depth, length and age. Different numbers of membership functions are defined for each factor according to its distribution. The proposed SHFL model takes only 95 rules as compared to the traditional mamdani fuzzy logic method that requires 1225 rules. It is very hard and time consuming for experts to design 1225 rules accurately and precisely. Further, we proposed a Do-it-Yourself (DIY) system for the proposed SHFL method. The purpose of the DIY system is that one can design the FIS model according to his or her need. © 2019 by the authors.},
note = {4},
keywords = {},
pubstate = {published},
tppubtype = {article}
}
Costache, R.; Pham, Q. B.; Sharifi, E.; Linh, N. T. T.; Abba, S. I.; Vojtek, M.; Vojteková, J.; Nhi, P. T. T.; Khoi, D. N.
Flash-flood susceptibility assessment using multi-criteria decision making and machine learning supported by remote sensing and GIS techniques Journal Article
In: Remote Sensing, vol. 12, no. 1, 2020, ISSN: 20724292, (100).
@article{2-s2.0-85077496791,
title = {Flash-flood susceptibility assessment using multi-criteria decision making and machine learning supported by remote sensing and GIS techniques},
author = { R. Costache and Q.B. Pham and E. Sharifi and N.T.T. Linh and S.I. Abba and M. Vojtek and J. Vojteková and P.T.T. Nhi and D.N. Khoi},
url = {https://www.scopus.com/inward/record.uri?eid=2-s2.0-85077496791&doi=10.3390%2fRS12010106&partnerID=40&md5=7b5f9bedb641ab5a52381b5e60c3b3c2},
doi = {10.3390/RS12010106},
issn = {20724292},
year = {2020},
date = {2020-01-01},
journal = {Remote Sensing},
volume = {12},
number = {1},
publisher = {MDPI AG},
abstract = {Concerning the significant increase in the negative effects of flash-floods worldwide, the main goal of this research is to evaluate the power of the Analytical Hierarchy Process (AHP), fi (kNN), K-Star (KS) algorithms and their ensembles in flash-flood susceptibility mapping. To train the two stand-alone models and their ensembles, for the first stage, the areas affected in the past by torrential phenomena are identified using remote sensing techniques. Approximately 70% of these areas are used as a training data set along with 10 flash-flood predictors. It should be remarked that the remote sensing techniques play a crucial role in obtaining eight out of 10 flash-flood conditioning factors. The predictive capability of predictors is evaluated through the Information Gain Ratio (IGR) method. As expected, the slope angle results in the factor with the highest predictive capability. The application of the AHP model implies the construction of ten pair-wise comparison matrices for calculating the normalized weights of each flash-flood predictor. The computed weights are used as input data in kNN-AHP and KS-AHP ensemble models for calculating the Flash-Flood Potential Index (FFPI). The FFPI also is determined through kNN and KS stand-alone models. The performance of the models is evaluated using statistical metrics (i.e.; sensitivity; specificity and accuracy) while the validation of the results is done by constructing the Receiver Operating Characteristics (ROC) Curve and Area Under Curve (AUC) values and by calculating the density of torrential pixels within FFPI classes. Overall, the best performance is obtained by the kNN-AHP ensemble model. © 2019 by the authors.},
note = {100},
keywords = {},
pubstate = {published},
tppubtype = {article}
}
Mehdizadeh, S.; Mohammadi, B.; Pham, Q. B.; Khoi, D. Nguyen; Linh, N. T. T.
In: Measurement: Journal of the International Measurement Confederation, vol. 165, 2020, ISSN: 02632241, (24).
@article{2-s2.0-85086829026,
title = {Implementing novel hybrid models to improve indirect measurement of the daily soil temperature: Elman neural network coupled with gravitational search algorithm and ant colony optimization},
author = { S. Mehdizadeh and B. Mohammadi and Q.B. Pham and D. Nguyen Khoi and N.T.T. Linh},
url = {https://www.scopus.com/inward/record.uri?eid=2-s2.0-85086829026&doi=10.1016%2fj.measurement.2020.108127&partnerID=40&md5=1f79245e945c2a73c4ae633755320ee2},
doi = {10.1016/j.measurement.2020.108127},
issn = {02632241},
year = {2020},
date = {2020-01-01},
urldate = {2020-01-01},
journal = {Measurement: Journal of the International Measurement Confederation},
volume = {165},
publisher = {Elsevier B.V.},
abstract = {Soil temperature (ST) as a vital variable of soil plays a key role in agriculture products, surface energy transactions, soil moisture balance, etc. In developing countries like Iran, access to the ST data may be limited. Hence, estimating this parameter by an appropriate alternative approach is of great importance. Two novel hybrid models are developed in this study based on Elman neural network (ENN) coupled with gravitational search algorithm (GSA) and ant colony optimization (ACO) for improving the daily ST estimation at various soil depths (i.e.; ENN-GSA and ENN-ACO). In fact, both the optimization algorithms including the GSA and ACO were applied to train the parameters of ENN. To achieve this, the daily data from two stations, namely the Isfahan and Rasht located in Iran were employed during 1998–2017. The classical ENN and hybrid ENN-GSA and ENN-ACO models are developed using the other meteorological parameters under eleven different scenarios. The results illustrated that the proposed hybrid models outperformed the classical ENN for estimating the daily ST of the studied locations at different depths; however, the hybrid ENN-GSA was the best-performing model at the studied stations and whole the soil depths. In addition, all the standalone and hybrid models illustrated the highest accuracy under full-input pattern. © 2020 Elsevier Ltd},
note = {24},
keywords = {},
pubstate = {published},
tppubtype = {article}
}
2019
Pham, Q. B.; Abba, S. I.; Usman, A. G.; Linh, N. T. T.; Gupta, V.; Malik, A.; Costache, R.; Vo, N. D.; Tri, D. Q.
Potential of Hybrid Data-Intelligence Algorithms for Multi-Station Modelling of Rainfall Journal Article
In: Water Resources Management, vol. 33, no. 15, pp. 5067-5087, 2019, ISSN: 09204741, (47).
@article{2-s2.0-85075940058,
title = {Potential of Hybrid Data-Intelligence Algorithms for Multi-Station Modelling of Rainfall},
author = { Q.B. Pham and S.I. Abba and A.G. Usman and N.T.T. Linh and V. Gupta and A. Malik and R. Costache and N.D. Vo and D.Q. Tri},
url = {https://www.scopus.com/inward/record.uri?eid=2-s2.0-85075940058&doi=10.1007%2fs11269-019-02408-3&partnerID=40&md5=18d0a392f3b63a45b94754dcde39f9a2},
doi = {10.1007/s11269-019-02408-3},
issn = {09204741},
year = {2019},
date = {2019-01-01},
journal = {Water Resources Management},
volume = {33},
number = {15},
pages = {5067-5087},
publisher = {Springer},
abstract = {One of the most challenging tasks in rainfall prediction is designing a reliable computational methodology owing the random and stochastic characteristics of time-series. In this study, the potential of five different data-driven models including Multilayer Perceptron (MLP), Least Square Support Vector Machine (LSSVM), Neuro-fuzzy, Hammerstein-Weiner (HW) and Autoregressive Integrated Moving Average (ARIMA) were employed for multi-station (Hien; Thank My; Hoi Khanh; Ai Nghia and Cai Lau) prediction of daily rainfall in the Vu Gia-Thu Bon River basin in Central Vietnam. Subsequently, hybrid ARIMA-MLP, ARIMA-LSSVM, ARIMA-NF and ARIMA-HW models were also utilized to predict the daily rainfall at these stations. The results were evaluated in terms of widely used performance criteria, viz.: determination coefficient (R2), root mean square error (RMSE), mean absolute error (MAE) and correlation coefficient (CC). Besides, the Taylor diagram is also used to examine and compare the similarity between the observed and predicted rainfall. The quantitative analysis indicated that the HW model increased the prediction accuracy by 5%, 3% and 2% at Hien, Ai Nghia and Cau Lau stations, respectively, compared to the other models. Likewise, the NF model increased the prediction accuracy at Thanh My and Hoi Khanh stations in contrast to the other models in terms of the mean absolute error. Also, the results of hybrid ARIMA-NF and ARIMA-HW models showed the best performance in terms of predictive skills and verified to increase the prediction accuracy in comparison to the single models. © 2019, Springer Nature B.V.},
note = {47},
keywords = {},
pubstate = {published},
tppubtype = {article}
}
Sharma, C.; Ojha, C. S. P.; Shukla, A. K.; Pham, Q. B.; Linh, N. T. T.; Fai, C. M.; Loc, H. H.; Dung, T. D.
Modified approach to reduce GCM bias in downscaled precipitation: A study in Ganga River Basin Journal Article
In: Water (Switzerland), vol. 11, no. 10, 2019, ISSN: 20734441, (4).
@article{2-s2.0-85073193920,
title = {Modified approach to reduce GCM bias in downscaled precipitation: A study in Ganga River Basin},
author = { C. Sharma and C.S.P. Ojha and A.K. Shukla and Q.B. Pham and N.T.T. Linh and C.M. Fai and H.H. Loc and T.D. Dung},
url = {https://www.scopus.com/inward/record.uri?eid=2-s2.0-85073193920&doi=10.3390%2fw11102097&partnerID=40&md5=84285f72e10b447f0d7cf8d9b710e6ae},
doi = {10.3390/w11102097},
issn = {20734441},
year = {2019},
date = {2019-01-01},
journal = {Water (Switzerland)},
volume = {11},
number = {10},
publisher = {MDPI AG},
abstract = {Reanalysis data is widely used to develop predictor-predictand models, which are further used to downscale coarse gridded general circulation models (GCM) data at a local scale. However, large variability in the downscaled product using different GCMs is still a big challenge. The first objective of this study was to assess the performance of reanalysis data to downscale precipitation using different GCMs. High bias in downscaled precipitation was observed using different GCMs, so a different downscaling approach is proposed in which historical data of GCM was used to develop a predictor-predictand model. The earlier approach is termed "Re-Obs" and the proposed approach as "GCM-Obs". Both models were assessed using mathematical derivation and generated synthetic series. The intermodal bias in different GCMs downscaled precipitation using Re-Obs and GCM-Obs model was also checked. Coupled Model Inter-comparison Project-5 (CMIP5) data of ten different GCMs was used to downscale precipitation in different urbanized, rural, and forest regions in the Ganga river basin. Different measures were used to represent the relative performances of one downscaling approach over other approach in terms of closeness of downscaled precipitation with observed precipitation and reduction of bias using different GCMs. The effect of GCM spatial resolution in downscaling was also checked. The model performance, convergence, and skill score were computed to assess the ability of GCM-Obs and Re-Obs models. The proposed GCM-Obs model was found better than Re-Obs model to statistically downscale GCM. It was observed that GCM-Obs model was able to reduce GCM-Observed and GCM-GCM bias in the downscaled precipitation in the Ganga river basin. © 2019 by the authors.},
note = {4},
keywords = {},
pubstate = {published},
tppubtype = {article}
}